下载中心
优秀审稿专家
优秀论文
相关链接
摘要
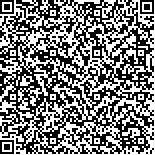
目前基于深度学习的云检测方法,受训练样本限制,算法难以推广及应用。为了快速实现针对多种传感器的高精度的云检测,Sun等(2020)提出统一样本云检测方法。基于AVIRIS高光谱样本库模拟出待检测传感器的云和晴空地表像元,将模拟得到的多光谱样本数据输入到BP神经网络中进行逐像元分类,生成云检测模型,实现Landsat 8 OLI等宽光谱传感器较高精度的云检测。该方法基于统一样本模拟出不同传感器的样本像元库,适用于多种传感器的云检测。由于Landsat 8 OLI波段较多,波谱范围覆盖宽,容易实现云的高精度识别。为了进一步提高其在光谱范围较窄的GF-6 WFV数据上的云检测应用精度,在模拟出的样本库中加入GF-6 WFV数据典型高亮地表像元。通过目视解译对云检测结果进行精度验证,结果表明,该算法利用可见光和近红外通道的遥感数据可以高精度的识别出植被、水体、建筑、裸地等地表类型上空的厚云、碎云和薄云。改进后的云检测算法,云像元平均正确率达到88.40%,在高亮地表上空云像元正确率达到87.40%,在不同地表类型上空的云像元平均正确率为92.60%。结果表明,加入高反射率地物的算法可以利用有限波段实现云和地表的高精度分离。
关键词:
云检测 GF-6 WFV数据 高光谱像元库 亮地表 深度学习算法The unified sample cloud detection method proposed by Sun et al. based on the AVIRIS hyperspectral sample database simulates the cloud and clear sky surface pixels of the sensor to be detected. The method also inputs the simulated multi-spectral sample data into BP neural network for pixel-by-pixel classification to generate cloud detection models. This method can realize high-precision cloud detection of Landsat 8 OLI and other wide spectrum sensors. This method, which simulates the sample pixel libraries, is suitable for cloud detection of various sensors. Given that the Landsat 8 OLI sensor has more bands, the spectrum covers a wide range, easily facilitating high-precision cloud identification.In this paper, an improved cloud detection algorithm is proposed. Owing to the narrow spectral range of GF-6 WFV data and the lack of cloud-sensitive bands such as 1.38 μm, 1.65 μm, and thermal infrared bands, cloud identification accuracy in high reflectivity areas is low. The cloud and clear sky image metadata simulated based on the unified sample pixel database have a weak ability to identify clouds and bright surfaces, and realizing stable and high-precision cloud identification of this type of satellite is impossible. To further improve the application precision of cloud detection on GF-6 WFV data with a narrow spectral range, GF-6 WFV data typical highlighted surface pixels were added into the simulated sample base to realize cloud detection of GF-6 WFV data with high precision.The main content of the improved unified sample cloud detection algorithm is as follows. (1) GF-6 WFV multi-spectral data simulation. This paper simulates the apparent reflectance of the corresponding band of GF-6 WFV data by the weighted synthesis of the band of AVIRIS hyperspectral data. (2) BP deep learning cloud detection model. In the simulated GF-6 WFV data sample base, typical highlighted surface samples, such as bare land, buildings, and snow, in the GF-6 WFV data are added. The apparent reflectance of each band of the improved data pixel in the sample base is taken as the input vector. The inductive ability of the neural network is used to learn cloud detection rules, generate a cloud detection model, and conduct cloud detection experiments.The accuracy of cloud detection results was verified by visual interpretation. The artificial labeled cloud area was taken as the reference truth value, and the cloud detection results of the algorithm were compared with the reference truth value on a per-pixel basis. By constructing an error matrix, the precision of cloud inspection results of the proposed algorithm is calculated and verified. With the improved cloud detection algorithm, the average correct rate of cloud pixels reaches 0.884 and 0.874 for cloud pixels over the highlighted land surface and 0.926 for cloud pixels over different land surface types.The results show that the algorithm can accurately identify the thick clouds, broken clouds, and thin clouds over vegetation, water, buildings, and bare land with high precision by using the remote sensing data of visible and near-infrared channels. The algorithm of adding high-reflectivity ground objects can use limited bands to achieve high-precision separation of clouds and ground.