下载中心
优秀审稿专家
优秀论文
相关链接
摘要
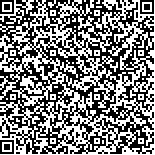
高分辨率遥感影像地物复杂,分类难度大,而深度学习方法可以提取地物更多更深层次的特征信息,适用于高分辨率遥感影像的地物分类。本文研究对高分辨率影像中不透水地面、建筑、低矮植被、树、车辆等地物的高精度分类。结合遥感多地物分类的特点,以DeepLab v3+网络模型为基础,提出E-DeepLab网络模型。主要改进为:(1)改进编码器和解码器的结合方式,使用简洁有效的加成连接方式。(2)缩小单次上采样倍数,增加上采样层,提高编码器与解码器连接的紧密性。(3)使用改进的自适应权重损失函数,自动调节地物损失权重。同时根据数据特点,提出结合DSM、NDVI数据等多通道训练方式。使用两个地区数据进行实验,结果表明,两地区精度均明显优于原始DeepLab v3+模型和其他相关模型,Potsdam地区总体提取精度达到93.2%,建筑物提取精度达到97.8%,Vaihingen地区总体提取精度达到90.7%,建筑物提取精度达到96.3%。目视对比分类图和标准标记图,两者具有高度的一致性。本文所提出的E-DeepLab网络在高分辨率遥感影像地物高精度提取和分类中有较好的应用价值。
High-resolution remote sensing images are complex and difficult to classify. Deep learning methods can extract more and deeper information of the features, which is suitable for the classification of high-resolution remote sensing images. This paper studies the high-precision classification of impervious ground, buildings, low vegetation, trees, vehicles, and other features in high-resolution images. An E-DeepLab network model is proposed by combining the characteristics of remote sensing multiground feature classification based on DeepLab v3+ network model. The main improvements are as follows: (1) Improving the combination of encoder and decoder modules and using a simple and effective addition connection method. (2) Reducing the single upsampling multiple, increasing the upsampling layer, and improving the tightness of the connection between the encoder and the decoder. (3) Using the improved adaptive weight loss function to automatically adjust the weight of losses. In accordance with the characteristics of the data, a multichannel training method combining digital surface model and normalized difference vegetation index data is proposed. Using the data from the two regions to conduct experiments, the overall extraction accuracy in Potsdam region reaches 93.2%, the extraction accuracy in buildings reaches 97.8%, the overall extraction accuracy in Vaihingen region reaches 90.7%, and the accuracy of building extraction reaches 96.3%. The accuracy of the two regions is significantly better than the original DeepLab v3+ model and other related models. The visual effect of the classification results is extremely close to the standard map by comparing the classification map and the standard marker map. Results show that the E-DeepLab network has good application value in the feature extraction and classification of high-precision remote sensing images.