下载中心
优秀审稿专家
优秀论文
相关链接
摘要
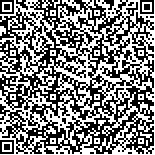
定量遥感是从原始遥感观测信息中定量推算或反演出地学参量的理论与方法。传统定量遥感主要基于模型驱动,强调通过数学或物理模型完成推算和反演。随着人工智能技术的发展和普及,数据驱动的方式也逐渐受到广泛关注,其强调的是通过机器学习等方式挖掘遥感观测数据中所包含的信息,完成地学参量的定量反演。在强大计算能力的支持下,数据驱动的方法在定量遥感的多个领域都取得了可喜的成就,但其也具有弱化物理规律及因果关系的缺点。在此背景下,耦合物理规律和机器学习,发展模型和数据共同驱动的反演框架,开始成为新的研究热点。以机器学习辅助物理模型,或以物理规律约束机器学习的研究逐步展开,并初见成效。然而,仍面临着较大的挑战,联合模型的不确定性、泛化性、可迁移性,以及小样本情况下的联合建模等都是急需解决的问题。未来,数据驱动和模型驱动的深度耦合将是待突破的难点和重点。
Quantitative remote sensing is a technique for quantitatively inferring or inverting earth environmental variable from the original remote sensing observations, it is an important step to turn electromagnetic wave signals into easy-to-understand information about surface environment. Traditional quantitative remote sensing methods are mainly model-driven, emphasizing inversion through mathematical or physical models. With the development and popularization of artificial intelligence technology, data-driven methods have gradually received widespread attention. It emphasizes the use of machine learning methods to mine the information contained in remote sensing observation data to achieve the quantitative inversion of geophysical parameters. With the support of powerful computing capacity, the data-driven method has achieved gratifying achievements in many fields of quantitative remote sensing. This article systematically summarizes the principles, characteristics, and applications in quantitative remote sensing field of different types of data-driven models, including regression algorithms, regularization methods, instance-based algorithms, decision tree, Bayesian methods, kernel based algorithms, genetic algorithms, ensemble learning, artificial neural network, and deep learning. Though data-driven models show satisfying retrieval performance in multiple fields, its drawbacks of ignoring the laws of physics and lack of causality have also brought resistance to its development. In this context, coupling the laws of physics and machine learning to develop an inversion framework driven by both models and data has become a new research hotspot. Some pioneering researches have already achieved delightful performance through using machine learning to assist physical models or restricting machine learning with physical laws. Using machine learning techniques to optimize the systematic basis, the sub-model, and the model parameters largely improve the performance of model-driven methods. Meanwhile, integrating physics knowledge into machine learning models through adjusting the training data, modifying the loss function, and constraining the solution space also benefit the improvement of data-driven models. However, there are still great challenges to be broken through. Physical models contain complex mechanisms and rich knowledge, current fusion of data-driven and model-driven methods are quite shallow with very limited amount of physical knowledge being used. A deeper coupling strategy is worth exploring in the future. Besides, the uncertainty, generalization, and transferability of the joint model have not been scientifically evaluated currently, to which attention should be paid. Finally, there are many cases when the training samples were very difficult to obtain, therefore, the applicability of the joint model in the case of small samples is also a problem that needs to be solved urgently. The deep, robust, and generalizable coupling of data-driven and model-driven models is expected in the future.