下载中心
优秀审稿专家
优秀论文
相关链接
摘要
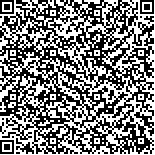
深度学习在高光谱图像处理领域的研究应用不断深入发展,基于深度学习的高光谱图像分类达到了较高的分类精度。目前的分类模型多利用高光谱的图谱特征,但对光谱的诊断性特征及先验信息利用不足,对空谱特征提取过程难以实现有效协同,因而导致分类类别即类内分类不够精细。为了解决以上问题,本文提出一种以多标签数据为输入的共生神经网络模型,在高光谱图谱特征提取的基础上融合光谱诊断特征,实现相对含水量反演及精细分类。首先,通过构建一种新的红边斜率光谱指数实现高光谱图像相对含水量的表征,利用本文提出的自适应分级算法完成相对含水量反演并建立对应的等级标签,与地物种类标签共同构成多标签高光谱数据集。然后,构建共生神经网络架构及内部变维特征提取模块,利用多标签数据提取高光谱图像中空间、光谱和相对含水量的融合特征,提高深度模型对不同含水量地物的区分能力和对所提取特征的协同表达能力,降低模型的复杂度与计算量,完成基于多标签数据集的相对含水量反演引导分类的过程,在扩大传统类间距离的基础上进一步扩大类内距离,从而实现高光谱图像的精细分类。最后,使用实验室采集数据和4个公开的高光谱数据集Lopex、Indian Pines、Pavia University和Salinas进行实验验证。结果表明,本文提出的红边斜率光谱指数可以有效表征地物的相对含水量信息;相对含水量反演引导的分类模型对类内分类精度有较明显的提升,对总体分类结果有一定的改善;与其他机器学习和深度学习分类算法相比,本文算法取得了较好的分类结果,提高了深度分类模型的分类性能和精细程度,实现了精细分类。
The application of deep learning methods in the hyperspectral research field has been developed continuously, and hyperspectral image classification models based on deep learning methods achieve high classification accuracy compared with others. Current classification models mostly use hyperspectral graphic-spectral features but lack diagnostic features and prior information, which makes it difficult to extract spectral-spatial features collaboratively and implement refined classification further. The results are not refined for intraclass classification. To solve the abovementioned issues, we proposed a Symbiotic Neural Network (SNN) for hyperspectral refined classification, which regards a multilabeled data set as input. SNN can fuse spectrum diagnostic features with graphic-spectral features to retrieve relative water content and implement refined classification simultaneously. First, we proposed a new spectral index, Red Edge Sloped (RES), to characterize the relative water content and used RES to label the relative water content on all the objects in data sets via an adaptive grading algorithm. We then built a multilabeled data set with original object labels. Second, we established the SNN architecture and dimensionality-varied feature extraction module to extract fusion information of space, spectra, and relative water content in hyperspectral data, which was able to improve the cooperative expression capability for features and the discrimination capability for the water content of various ground objects. It was also able to reduce the structural complexity and amount of computation of the deep model. Moreover, the dimensionality-varied module was able to extract more accurate and abstract features at a high level and improved the extensibility to build a deeper network. After the aforementioned progress, we implemented hyperspectral image refined classification with the guide of relative water content retrieval by using the SNN and completed interclass and intraclass classification simultaneously. On the basis of the relative water content retrieval in the classification, the interclass and intraclass distances can be further expanded over traditional classification methods. Experiments were conducted using one hyperspectral data set collected in the laboratory and four open hyperspectral data sets, namely, Lopex, Indian Pines, Pavia University, and Salinas, to validate the effectiveness of RES and the superiority of the SNN model. The experimental results demonstrated that the RES index proposed in this paper was able to represent the relative water content of objects in data effectively. The classification accuracy and discrimination capability for the water content of SNN were improved evidently with the guide of relative water content retrieval. In addition, we compared SNN with other state-of-the-art methods, and SNN obtained higher classification accuracy. Therefore, the SNN model proposed in this paper, which implements refined classification with the guide of relative water content retrieval, can enhance the feature extraction capability of the model for hyperspectral image classification and improve the classification performance effectively.