下载中心
优秀审稿专家
优秀论文
相关链接
摘要
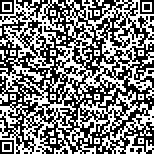
机器学习方法近年来取得突破进展,其遥感应用从目标识别和地物分类领域,发展到定量化反演的多个领域。气溶胶定量遥感因其机理复杂,反演参数的种类和精度受到限制,机器学习为气溶胶遥感带来了新的研究和应用技术手段。本文汇总现有研究进展将气溶胶机器学习方法归纳为卫星遥感反演气溶胶光学厚度AOD(Aerosol Optical Depth)、卫星遥感反演其他气溶胶参数、卫星遥感反演颗粒物浓度(PMx)、地基气溶胶遥感4类。结合作者研究工作,通过分析讨论,归纳机器学习用于气溶胶定量遥感的条件为:(1)物理模型无法使用;(2)已有模型卫星产品精度低;(3)已有模型精度高但计算速度低。从应用的角度来说,可以借助于更多的具有相关性的输入信息,发挥机器学习在反演产品种类、反演精度、计算效率等方面的优势;而对定量遥感来说,应该同时重视挖掘遥感数据本身的信息来提高反演能力,并通过误差分析等手段反馈对遥感机理的理解,使机器学习与遥感机理研究相互促进。
Machine learning methods have made breakthroughs in recent years, and their remote sensing applications have developed from remote sensing image recognition and classification to many fields in quantitative retrievals. Owing to their complicated mechanism in quantitative aerosol remote sensing, the types and accuracy of retrieved parameters are limited. Machine learning introduces new research and application techniques to aerosol remote sensing. Existing aerosol retrieval machine learning methods are summarized into four categories: satellite aerosol optical depth remote sensing, other aerosol parameters’ satellite remote sensing, particulate matter concentration, and ground-based aerosol remote sensing. In consideration of the authors’ research, through analysis and discussion, the conditions for machine learning to be used for aerosol quantitative remote sensing are summarized as follows: (1) Physical models could not be utilized. (2) Existing models have low accuracy. (3) Existing models have low computational efficiency. From the perspective of application, relevant inputs can be used to utilize machine learning to improve retrieved product types, retrieval accuracy, and calculation efficiency. For the quantitative remote sensing research field, how to mine information from remote sensing data should also be paid attention to improve the retrieval capability. Machine learning can also feedback the understanding of remote sensing mechanisms through error analysis, such that machine learning and remote sensing mechanism research can promote each other.