下载中心
优秀审稿专家
优秀论文
相关链接
摘要
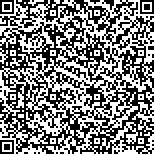
株高和地上生物量AGB(Above-Ground Biomass)是作物长势监测的重要指标,因此快速获取这些信息对指导田间管理具有重要意义。本研究通过无人机搭载高清数码相机分别获取马铃薯5个生育期的影像数据,地面实测株高H(heigh)和AGB以及地面控制点GCPs(Ground Control Points)的三维空间坐标。首先,利用试验区域的影像数据结合GCPs的位置信息从生成的数字表面模型DSM(Digital Surface Model)中提取出马铃薯的株高(Hdsm)。其次,选取26种植被指数和H、Hdsm组成新的数据集与AGB作相关性分析,筛选出相关性较高的前7个植被指数同Hdsm作为估算马铃薯AGB的输入参数。然后,使用MLR(Multiple Linear Regression)、SVM(Support Vector Machine)和ANN(Artificial Neural Network)方法分别基于植被指数、植被指数和Hdsm构建马铃薯多生育期AGB估算模型,对不同估算模型进行比较分析,从而选择出AGB估算的最佳模型。结果表明:基于DSM提取的Hdsm与实测株高H高度拟合(R2=0.86,RMSE=6.36 cm,NRMSE=13.42%);各生育期基于3种回归技术均以植被指数融入Hdsm构建的模型精度最高,估算能力最强;各生育期利用MLR方法构建的AGB估算模型效果最佳,其次为SVM-AGB估算模型,而ANN-AGB估算模型效果最差。该研究可为马铃薯AGB快速、无损监测提供科学参考。
Plant height and Above-Ground Biomass (AGB) are important agronomic parameters for crop growth monitoring. Therefore, efficiently and timely acquire this information of potato plant is important for guiding farmland production management. Traditionally, manual actual surveys are time-consuming, laborious and destructive, and fail to meet the modern needs of smart agriculture. With the advancement of science and technology, remote sensing technology has attracted people’s attention for its advantages of non-destructive, high-throughput, and rapid acquisition of phenotype information of ground objects. Compared with satellite, aerial and ground remote sensing, UAV remote sensing technology is widely popularized in precision agriculture monitoring due to its strong mobility, simple operation, low operating cost, and the ability to obtain high-resolution digital orthophotos under the cloud. In this study, the UAV equipped with high-definition digital camera was used to obtain the image data of potato with budding periods, tuber formation period, tuber growth period, starch accumulation period and maturity period, and the height (H) and AGB of potato plant on the ground were measured, and the longitude, dimension and height of Ground Control Points (GCPs) were obtained by Global Positioning System (GPS) from March to July 2019. Firstly, the Digital Surface Model (DSM) was generated by structure from motion algorithm based on the image data of the experimental area and the location information of GCPs, and the Hdsm (potato plant height) of each growth period was extracted based on DSM. Then, combining 26 image indices with better performance in AGB monitoring based on the digital number value of the image, crop height of field survey by ruler (H) and crop height extracted based on DSM difference calculation (Hdsm) into a new data set. The first 7 indices and Hdsm based analyzing the correlation between these parameters (26 vegetation indices, H and Hdsm) and AGB were screened to construct the AGB estimation model of five growth periods. Finally, in order to further increase the variance of the different model, Multiple Linear Regression (MLR), Support Vector Machine (SVM) and Artificial Neural Network (ANN) are selected to build the AGB estimation model based on the sensitivity parameters. Through the quantitative analysis of the model, the optimal estimation model is selected for each growth period to monitor crop conditions. The results showed that: the extracted plant height (Hdsm) is fitted with the measured plant height (R2=0.86, RMSE=6.36cm, NRMSE=13.42%); the AGB estimation model was constructed by three different modeling methods in each growth period, in which the model by integrating with Hdsm into vegetation indices was better; it is found that the effect of MLR model (R2 =0.61, 0.74, 0.77, 0.72 and 0.60) with incorporating the Hdsm into image indices in each growth period to estimate AGB is better than that of SVM (R2=0.60, 0.69, 0.73, 0.69 and 0.58) and ANN (R2=0.56, 0.67, 0.71, 0.65 and 0.55). The results of this research help solve the problem of monitoring AGB in the traditional way and provide reference for real-time monitoring of potato growth and yield prediction accurately.