下载中心
优秀审稿专家
优秀论文
相关链接
摘要
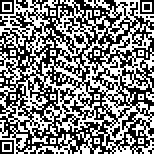
高时空分辨率的全球多类别土地覆盖数据对于地球系统的生物化学循环、气候变化等研究至关重要。目前公开的数据产品中,较高空间分辨率的全球多类别土地覆盖产品仅提供单一或短时期的数据,而全球逐年土地覆盖产品往往只有单一土地覆盖类型,难以从较长时间跨度上反映精细地物的年际变化。本文借助Google Earth Engine平台,利用现有多套全球土地覆盖产品、Landsat卫星系列影像、以及大量人工目视解译样本,结合多数据融合、时序变化检测和机器学习等的方法,研制了一套2000年—2015年全球30 m分辨率的逐年土地覆盖变化数据集AGLC-2000-2015(Annual Global Land Cover 2000-2015)。基于AGLC-2000-2015数据集,本文选择性分析了3个典型区域(中国珠江三角洲地区、青藏高原色林错湖区和亚马逊热带雨林区)的土地覆盖年际变化。结果显示,AGLC-2000-2015数据集达到了较高的精度水平:基准年份产品(AGLC-2015)的总体精度(OA)为76.10%,Kappa系数为0.72,显著优于现有30 m分辨率的全球土地覆盖产品Globeland 30(OA = 63.49%,Kappa = 0.58)、FROM-GLC(OA = 61.41%,Kappa = 0.55)和GLC-FCS30(OA = 63.46%,Kappa = 0.57);年际间分类模型的总体精度和Kappa系数分别为84.10%和0.81,在各大洲的平均总体精度均超过80.00%,表明该模型在全球多类别土地覆盖分类中表现良好。3个典型区域的土地覆盖变化分析显示,中国珠江三角洲地区城市扩张趋势明显(195.96 km2/a),其增量主要来源于耕地(84.88%);青藏高原色林错湖泊对于气候变暖响应明显,湖区面积呈扩大趋势(17.95 km2/a),湖面北岸扩张最为明显;亚马逊热带雨林南部区域毁林造田趋势明显,15 a间森林面积减少46356.53 km2,其中大部分转化为农田(39621.29 km2)。上述结果表明:AGLC-2000-2015数据集能够有效反映全球陆地区域在30 m空间分辨率下的地表覆盖分布及年际间的动态演化,为地表陆面过程研究和相关应用提供可靠的数据支撑。
High-spatiotemporal-resolution global multi-class land cover data play a critical role in the studies of biogeochemical cycles of the Earth system and global climate change. Among the existing open products, higher-resolution global multi-class land cover data are available for a single or short period of time, while annual ones have limited class, preventing longer-term analysis of land cover change in fine spatial detail. Therefore, long time, high spatial resolution and high temporal frequency global land cover are needed.In this paper, with the support of the Google Earth Engine platform, we proposed a method composed of data fusion, change detection and machine learning based on existing global land cover maps in 2015, Landsat imagery and samples after manual interpretation to develop annual global land cover data (Annual Global Land Cover-2000-2015) from 2000 to 2015 at 30 m resolution. Based on AGLC-2000-2015 data, we selectively analyzed dynamic change of land cover in three typical regions (the Pearl River Delta of China, Selin Co lake of Tibetan Plateau and the Amazon rainforest of Brazil).Our results show relatively high accuracy of AGLC-2000-2015. Mean overall accuracy and Kappa coefficient of global land cover product for the year 2015 (AGLC-2015) are 76.10% and 0.72, respectively. The accuracies are considerably higher than that of existing global land covet products at 30m resolution, such as Globeland30 (OA=63.49%, Kappa=0.58), FROM-GLC (OA=61.41%, Kappa=0.55), and GLC-FCS30 (OA=63.46%, Kappa=0.57). The overall accuracy and Kappa coefficient of the Random Forest classification model are 84.10% and 0.81, respectively. In addition, the mean overall accuracy of Random Forest classification model at continental scale is more than 80.00%, showing that this model has good performance on global multi-class land cover mapping.We analyzed the land cover changes for the 2000—2015 period in three selected regions. Urban area has increased substantially by an average of 195.96 km2 in the Pearl River Delta of China, with 85% of the newly developed impervious surface encroaching on cropland. Selin Co lake responses significantly to the warming climate with expanding at a rate of 17.95 km2, and the expansion is most pronounced in the north bank. Forest in the south part of Amazon has decreased by a total area of 46356.53 km2, most of which is converted to cropland, showing forest destruction for cropland.AGLC-2000-2015 can effectively reflect the distribution and annual change of global land cover classes at 30 m resolution from 2000 to 2015, providing fundamental data for research and application related to land surface processes.