下载中心
优秀审稿专家
优秀论文
相关链接
摘要
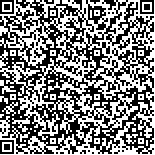
地表温度是陆面过程动态模拟、区域和全球变化分析等研究领域的重要参数,如何获得高时空分辨率地表温度数据一直是研究热点问题。选择河北省张家口市城郊区、山区两个区域作为试验研究区,在ERA5 0.1°分辨率地表温度订正基础上,构建随机森林降尺度模型以实现多层级分辨率的地表温度降尺度,并与Landsat 8 TIRS反演地表温度进行对比分析,以探讨再分析地表温度在不同分辨率、不同下垫面类型上的降尺度效果。结果表明:不同分辨率的降尺度结果都能够准确表达地表温度相对高低的空间分布特征,纹理精度显著提高,但降尺度误差随着空间分辨率提高而逐渐增大,城郊区和山区的降尺度均方根误差变化范围分别为1.16—1.79 ℃、1.61—2.49 ℃,地表温度高值与低值区域分别存在着低估和高估现象;随机森林降尺度模型中的参数重要性随尺度变化不大,总体表现为两个区域中植被指数NDVI的重要性都比较大,而海拔高度在山区区域对降尺度模型的影响更大。
Land Surface Temperature (LST) is an important parameter in many research fields such as dynamic simulation of land surface processes, regional and global change analysis. It has always been a hot research topic on how to obtain land surface temperature with higher spatio-temporal resolution. Due to the limitation in the availability of satellite imagery data with high spatial and temporal resolution simultaneously, LST downscaling from coarse spatial resolution data is an effective method. Besides LST retrieved from microwave channels or thermal infrared bands, reanalysis dataset can provide long time series of hourly land surface temperature. If the reanalysis LST can be downscaled to produce reliable products with higher spatial resolution or not needs to be further studied.To compare downscaling results at various resolutions from raw reanalysis LST, two different regions in Zhangjiakou, Hebei province were selected as the test areas that represents the urban-rural and the mountainous characteristics respectively. LST at 100 m spatial resolution was retrieved by using Landsat 8 OLI/TIRS data through Mono-Window (MW) algorithm, which was then upscaled to different resolutions of 200 m, 500 m, 1000 m, 2000 m, 5000 m and 10000 m respectively. ERA5 LST data at the resolution of 10000 m is corrected based on Landsat 8 LST, which is downscaled to 6 resolutions by constructing and applying the random forest model. Elevation and six remotely sensed indices including NDVI, MASVI, MNDWI, NMDI, NDBI, NDBSI calculating from the corresponding OLI spectral reflectance were taken as the random forest model parameters. LST downscaling precisions at different spatial resolution within various landcover regions were then evaluated and discussed by using the derived Landsat 8 LST as the reference, and the feature importance of seven land surface parameters in random forest models changed with scales were also analyzed by comparing Gini index.The maximum, minimum and average values of the corrected ERA5 LST and the reference Landsat 8 LST are close at the resolution of 10000 m, but the standard deviation is lower than that of reference LST. The downscaling results of different resolutions can accurately express the LST spatial distribution characteristics for the two experiment areas. As the spatial resolution changes from 5000 m to 100 m, the downscaled LST texture accuracy is significantly improved, however Root Mean Square Error (RMSE) gradually increases and the correlation between the downscaled and the reference LST decreases. RMSE grows from 1.16 to 1.79 ℃ and from 1.61 to 2.49 ℃ for the urban-rural area and the mountains area respectively. For the random forest downscaling model, features importance have no significant change at different resolutions, which to some degree indicates that the random forest model has a relatively stable scale invariant characteristic. NDVI shows higher importance affecting LST distribution in two test areas, and elevation is the most important parameter in mountainous area.The research results show that ERA5 LST and Landsat 8 LST have good consistency in the spatial distribution, which means ERA5 LST has the potentiality to be downscaled to express detailed land surface temperature at higher resolutions. While downscaling ERA5 LST to higher spatial resolution, larger underestimation and overestimation errors will occur in the high temperature area and low temperature area respectively.