下载中心
优秀审稿专家
优秀论文
相关链接
摘要
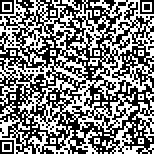
以光谱指数为趋势面因子的降尺度方法被广泛用于遥感地表温度尺度转换中,但面临构建的光谱指数难以凸显地表温度分布规律、浅层的统计模型难以精准刻画趋势面因子与地表温度之间的复杂关系的不足。为此,本文以Landsat 8 ARD 地表温度产品为降尺度对象,以Landsat 8 OLI原始数据为潜在趋势面因子,构建地表温度降尺度残差网络(LSTDRN)的深度学习模型;探索适用于Landsat 8地表温度产品空间降尺度的趋势面波段或组合,并在不同季节、不同地表类型下与经典传统方法TsHARP进行定量比较。结果表明:LSTDRN方法利用Landsat 8 OLI原始单波段作为趋势面因子就能有较好的降尺度效果,增加潜在趋势面因子的组合数量并不能提高降尺度效果。不同地表覆盖类型实验中,LSTDRN方法降尺度效果整体优于经典传统方法,且以近红外波段、红光波段和归一化植被指数为趋势面因子时,近红外波段降尺度效果定量评价表现最佳;不同地表覆盖类型的LSTDRN降尺度效果排序为:植被>建筑>水体,而经典传统方法则没有表现出明显的差异。不同季节实验中,LSTDRN方法在春夏冬3季的降尺度效果的定量评价表现明显好于经典传统方法,两类方法的秋季降尺度结果相当。因此,提出的LSTDRN对Landsat 8遥感地表温度产品具有较好的降尺度效果,整体优于经典传统方法且稳定性更强。
The downscaling method using spectral index as trend surface factor is widely used in remote sensing land surface temperature scale conversion. However, it is difficult to highlight the distribution of land surface temperature and describe the complex relationship between trend surface factor and land surface temperature in statistical model. Therefore, this paper constructs a Land Surface Temperature Downscaling Residual Network (LSTDRN) taking Landsat 8 ARD LST as downscaling objects and Landsat 8 OLI raw data as potential trend surface factors. The LSTDRN aims to explore the trend surface bands or combinations suitable for spatial downscaling of Landsat 8 land surface temperature products, and verify the spatiotemporal applicability of the model.In view of the strong nonlinear relationship fitting and feature extraction ability of deep learning, this paper proposes a LST downscaling model based on deep learning. In the training stage, the relationship model between the Landsat 8 ARD LST and all bands of Landsat 8 OLI (except band 9) is fitted at the low resolution level. The Huber loss function is used to minimize the residual between the prediction results and the label to realize the transformation residual constraint. Then the optimal model is obtained through iterative learning and parameter adjustment. In the test stage, the optimal model is applied at the high resolution level to obtain the final downscaling results according to the “scale invariant” hypothesis of land surface temperature downscaling. In addition to visual evaluation, the downscaling results and original LST data were scaled up to 100 m resolution for quantitative evaluation. After evaluating the downscaling effect of each band, the multi-trend surface factor downscaling experiment was carried out. Meanwhile, the deep learning method is compared with the classic traditional method TsHARP to compare the stability of different land surface types and different seasons.In LSTDRN, using the original single band of Landsat 8 OLI as the trend surface factor has a good downscaling effect, and increasing the number of potential trend surface factors can not improve the downscaling effect. In the experiments of different land cover types, the effect of deep learning method is better than that of traditional methods, and the best effect is when NIR band is the trend surface factor. The order of deep learning downscaling effect in quantitative evaluation of different land cover types is vegetation > building > water, however, there is no obvious difference between the traditional methods. In different season experiments, the downscaling effect in quantitative evaluation of deep learning method in spring, summer and winter is better than that of traditional classical methods, and the downscaling results of the two methods in autumn are similar. Therefore, the proposed LSTDRN has better downscaling effect on Landsat 8 remote sensing land surface temperature products, which is superior to the traditional classical methods and has stronger stability.The LSTDRN proposed in this study can make good use of Landsat 8 OLI single band data as trend surface factors for downscaling. With the increase of trend surface factors, the downscaling effect is not significantly improved. Compared with the classic traditional method TsHARP, the downscaling effect of deep learning method is less affected by land cover types and seasonal factors, and the difference of downscaling effect is rather small in different spatial-temporal conditions. The method has stronger stability, which is conducive to enhancing the applicability of downscaling research and promoting the application of high-quality land surface temperature data.