下载中心
优秀审稿专家
优秀论文
相关链接
摘要
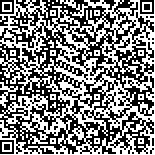
由于星载热红外传感器研发技术的局限性,单一传感器尚不能提供兼具高频次、高空间分辨率地表温度数据。协同其他遥感辅助数据,对低空间分辨率、高时间频次地表温度产品开展降尺度研究成为了解决这一难题的有效途径。然而由于现有地表温度降尺度方法未充分考虑不同地表状态参数对地表温度空间分异格局的尺度影响差异,降尺度后的地表温度数据在异质性景观区域存在精度较差和空间纹理不清晰的问题。鉴于此,本文以北京和张掖地区的8期MODIS地表温度产品为例,通过引入多尺度地理加权回归MGWR(Multiscale Geographically Weighted Regression)来分析归一化植被指数NDVI、数字高程模型DEM、坡度和经纬度对地表温度空间格局影响的尺度差异,提出一种针对MODIS地表温度产品的空间降尺度算法,并与TsHARP算法、多元线性回归算法、地理加权回归算法和随机森林回归算法进行定量对比。结果表明,基于MGWR模型的地表温度降尺度转换函数能够良好地揭示多种地表状态参数与地表温度间的不同作用关系,其中NDVI和坡度对地表温度分布具有全局影响,DEM和经纬度对地表温度呈现出了局域性作用。与4种代表性方法相比,基于MGWR算法降尺度后的100 m分辨率地表温度数据具有更好的空间纹理,在城镇和沙漠等温度异质性明显地区保障了清晰的景观纹理;另外,对于所选研究区的8期MODIS地表温度产品而言,利用MGWR算法降尺度后的地表温度均拥有更好的精度,在0—1 K误差级别下的面积占比均大于57%,均方根误差RMSE(Root-Mean-Square Error)均小于2.85 K,决定系数R2(coefficient of determination)均大于0.88。
Due to the limitations of thermal infrared technology, a single sensor cannot provide both high frequency and fine spatial resolution Land Surface Temperature (LST) data. For solving this problem, it becomes an effective way by conducting the spatial downscaling of LST product with low-resolution and high frequency in collaboration with other auxiliary data. However, the existing LST downscaling methods do not fully consider the scale effects of different biophysical parameters on the distribution of LST, which makes the accuracy and spatial distribution of the downscaled LST are inconsistent. In view of this, taking Beijing and Zhangye as two study areas, this paper proposed a kind of LST downscaling algorithm to sharpen the MODIS LST using Multi-scale Geographically Weighted Regression (MGWR) according to the analyse of effects of NDVI, DEM, slope, latitude, and longitude on LST heterogeneity. Furthermore, four kinds of LST downscaling methods (i.e., TsHARP algorithm, ML algorithm, GWR algorithm, and RF algorithm) were introduced in this paper for further comparison and validation. Results show that the constructed LST conversion function based on the MGWR reveals the actual interaction between various scale factors and LST at various spatial scales. NDVI and slope have global impacts on the LST, while DEM and geolocation present local impacts on the LST. Compared with the four referenced methods, the downscaled 100 m resolution LST based on the MGWR has better spatial textures and displays clear landscape features in heterogeneous areas such as deserts and towns. In addition, all images predicted by the MGWR algorithm showed better accuracy, in which the area proportion under the 0—1 K error level were all more than 57%, the root-mean-square error (RMSE) were all less than 2.85 K, and the coefficient of determination (R2) were all more than 0.88.