下载中心
优秀审稿专家
优秀论文
相关链接
摘要
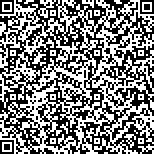
热红外遥感是监测地表温度的重要技术手段。然而,由于其易受云雾影响,热红外遥感反演地表温度存在大量的观测空白区域,严重影响地表温度产品的应用。近年来,机器学习算法的发展为实现地表温度无缝观测提供了新的技术手段。然而,基于机器学习的云雾覆盖像元重建方法与训练样本的数量和分布有着直接的联系,其应用条件在现有研究中还鲜有讨论。为深入探究重建过程中训练数据量和数值分布对重建精度的影响,本文选择基于随机森林的地表温度重建模型开展样本敏感性分析,采用美国MODIS陆地产品和欧洲第二代静止气象卫星(MSG)入射短波辐射产品重建不同训练样本集下的地表温度,并与真实地表温度数据比较,定量评估重建结果精度与样本数量和分布之间关系。研究结果表明:(1)地表温度重建精度随着训练样本数据量增加显著转好。(2)在数据量一定的情况下,随机采样方式因为具有空间代表性比区域取样方式具有更精确更稳定的精度,能将重建后的均方根误差降到2.1 K以下,相关系数达到0.93以上。即使数据量较小,随机取样方式的重建精度较稳定的特点使得模型对因数据量不足造成的重建精度降低的负面效应具有减弱作用。(3)进一步划分不同高程带和植被覆盖条件下的训练集,当训练集所处范围与重建集所处范围一致或者训练集覆盖范围越广时,重建精度越好。总体而言,上述研究成果为今后采用机器学习方法重建地表温度应用中训练样本选择以及获取高精度地表温度重建结果提供了重要的科学参考。
Land Surface Temperature (LST) represents integrated features of land atmosphere physical and dynamic processe, It is a key element in the fields of climate change, the land–atmosphere energy budget, and the global hydrological cycle, vegetation monitoring urban climate and environmental studies. Thermal infrared remote sensing is an important technique for monitoring LST. However, the Moderate Resolution Imaging Spectroradiometer (MODIS) data are severely contaminated by cloud cover, which limits the applications of LST products. In recent years, the development of machine learning algorithms provides a promising technique for the reconstruction of LST under clouds. However, the accuracy of the cloud cover pixel reconstruction method based on machine learning is directly related to the number and regional distribution of training samples. In order to quantitatively evaluate the impact of the number and regional distribution of training samples on the LST reconstruction accuracy, based on MODIS land products and Meteosat Second Generation (MSG) incident short-wave radiation products, the LST reconstruction model depending on random forest method to construct an LST linking model for LSTs and a range of influencing factors were fitted based on clear-sky observations, which was then applied to cloud-covered pixels to obtain an LST reconstruction, the proposed reconstruction model was applied to carry out the influence of different training samples on the reconstruction accuracy of LST. The results show that: (1) A visual comparison with daily LST observations from (MSG) incident short-wave radiation products indicated that the LSTs reconstructed using this method were representative of LST patterns resulting from the influence of key variables including solar radiation intensity, vegetation cover, and geographical factor. (2) The accuracy of LST reconstruction improves significantly with the increase of the amount of training sample data, and the reconstruction accuracy is also different in different seasons. When the amount of training data increases from 5% to 95%, there are seasonal differences between summer and autumn due to the differences in vegetation and solar radiation. The variation range of correlation coefficient and root mean square error in summer is larger than that in autumn. (3) The random sampling method has higher and stabler accuracy than the regional sampling method because of its spatial representativeness, which can reduce the root mean square error to less than 2.1 k and increase the correlation coefficient to more than 0.93. Even if the amount of data is small, the reconstruction accuracy with the random sampling method is relatively stable, the negative effect of the insufficient number of training samples on the reduction of reconstruction accuracy is weakened. (4) The training sets were divided according to different elevations and vegetation coverage ranges to reconstruct the LST, and the results showed that the reconstruction accuracy was better when the range of the training sets included the range of the reconstruction area, that is, when the training set contains enough data features, it has a satisfactory spatial representation. The research results show that the proposed reconstruction model has a strong potential to reconstruct LSTs under cloud-covered conditions, and can also accurately describe the spatial distributions of LST. It also can provide a reference for future machine learning methods to select appropriate training samples and reconstruct the LST with high accuracy.