下载中心
优秀审稿专家
优秀论文
相关链接
摘要
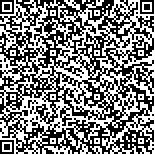
土壤水分不仅是陆面过程中重要的变量,同时也是全球水循环中的关键参数。为了获得高分辨率的土壤水分数据,本文将基于自适应窗口的土壤水分降尺度方法应用在闪电河流域,以1 km MODIS产品(地表温度和归一化植被指数)作为辅助数据,对9 km的SMAP被动微波土壤水分(SMAP土壤水分)数据进行降尺度,得到研究区1 km的降尺度土壤水分数据。利用地面站点实测土壤水分和机载被动微波土壤水分(机载土壤水分)对降尺度土壤水分和SMAP土壤水分进行了验证,并对辅助数据和降尺度方法本身展开分析以探讨降尺度过程中的不确定性来源。结果表明:(1)本文使用的基于自适应窗口的土壤水分降尺度方法能够有效地提高SMAP土壤水分的空间分辨率,在进一步丰富土壤水分分布细节变化信息的同时,还能够保留SMAP土壤水分的空间变化特征并与其保持值域一致。(2)3种基于像元尺度的土壤水分数据(机载土壤水分、SMAP土壤水分和降尺度土壤水分)与站点实测土壤水分之间的相关性并不高,这主要与点、面数据之间的空间匹配不一致、空间代表性不同以及有效验证的数据量有限有关。而与站点数据验证相比,降尺度土壤水分和SMAP土壤水分均和机载土壤水分数据相关性较好。(3)SMAP土壤水分与辅助数据之间的相关性比机载土壤水分与辅助数据之间的较高,而这两种土壤水分数据之间存在的这种偏差主要受到空间尺度、观测配置、参数反演算法和选用的辅助数据等因素的影响。(4)针对验证结果的不确定性,通过增加辅助数据或改变土壤水分估算模型结构进而修改降尺度模型的方式在本研究中并不能显著提高降尺度结果的精度,如何进一步提高降尺度精度仍是未来需要研究的重点。
Soil Moisture (SM) is not only an important variable in land surface processes, but also a key parameter in global water cycle. In this paper, the objectives are: (1) downscaling SMAP (Soil Moisture Active Passive) SM (SMAP SM) from spatial resolution of 9 km to 1 km, with the using of the auxiliary data from MODIS (Moderate-Resolution Imaging Spectroradiometer) products (land surface temperature and normalized difference vegetation index) by a downscaling method based on self-adaptive window in Shandian river basin; (2) validating the downscaled SM with the in-situ SM and the airborne passive microwave SM (airborne SM); and (3) analyzing the uncertainty caused by auxiliary data and SM estimated model in the downscaling process. The downscaling method used in this paper involves two steps. The SM model was established by using geographically weighted regression model between SMAP SM and the auxiliary data to calculate the 1-km estimated model SM (SMR). Then the 9-km residual (RC) generated by the SM estimated model is downscaled to 1-km spatial resolution (RF) by area-to-point kriging. Finally, the downscaled SM (SMF) is the sum of SMR and RF. It’s worth noting that to derive the robust downscaled SM, self-adaptive windows are adopted in these two steps. Visual assessment shows that the downscaling method can not only improve the spatial resolution of SMAP SM, but also retain the consistency between the spatial distributions of the downscaled SM and of the original SMAP SM. The validation results of the airborne SM, the SMAP SM and the downscaled SM against the in-situ SM are not satisfactory. On Sep 24, the correlation coefficient (R) between the three SM data and the in-situ SM are less than 0.5, and on Sep 26, the root mean squared errors (RMSE) are greater than 0.08 m3/m3. By analyzing these data, we found that the limited amount of valid data used in validation was one of the reasons for the poor validation. In addition, the different spatial representativeness and the inconsistent spatial matching of point-scale data and pixel-scale data are also the factors caused the uncertainty in the validation results. Compared with the in-situ SM, the SMAP SM and the downscaled SM have better correlations with the airborne SM. The RMSEs between the downscaled SM and the airborne SM are about 0.04 m3/m3, while the RMSEs between the SMAP SM and the airborne SM are less than 0.04 m3/m3. The correlation between the SMAP SM and auxiliary data (the absolute values of Rs are greater than 0.6) is higher than that between the airborne SM and the auxiliary data (the absolute values of Rs are less than 0.53). It can be seen that there are some differences between the SMAP SM and the airborne SM, which is mainly affected by different spatial scales, observation configurations, SM derived algorithms and auxiliary data using in algorithms of these two SM data. However, more studies are needed on the mechanism of the relationship between auxiliary data and SM in the downscaling process. By adding auxiliary data (land surface albedo) or changing the SM estimation model, the validated results of the downscaled SM against the airborne SM did not improve obviously. This is mainly because more auxiliary data and higher polynomials caused overfitting in the downscaling process, which will be still the focus of future research.