下载中心
优秀审稿专家
优秀论文
相关链接
摘要
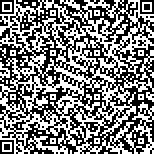
土壤水分在地表动植物生存、大气—地表间的能量和物质循环中承担着重要的角色,对水循环、气候变化、农业监测、生态环境、地质灾害等应用指标的大面积监测具有重要意义。但由于土壤类型、土壤结构条件、地形特征、植被环境以及人类活动等因素的影响,土壤含水量的分布存在空间异质性特点,对较大区域(例如流域尺度)的土壤水分分布进行监测仍然十分困难。本文以闪电河流域为例,利用中分辨率成像光谱仪(MODIS)反射率数据反演得到植被指数,并以相关植被指数VIs(Vegetation Indices)、地表温度LST(Land Surface Temperature)数据为输入参数,实测土壤水分数据为期望输出参数,发展了一种基于极端随机树的土壤水分反演方法。考虑到地表温度的不易测量性以及对区域土壤湿度监测的需求,本文使用短波红外转换反射率STR(Shortwave Infrared Transformed Reflectance)代替LST建立极端随机树模型,反演了覆盖闪电河流域的2° × 2°区域的土壤湿度图。实验结果表明:(1)输入参数使用LST时,基于极端随机树的土壤湿度反演模型表现较好,其均方根误差为0.054 m3m-3,相关系数为0.69,预测精度优于其他模型(支持向量机、随机森林);(2)输入参数使用STR时,预测结果的均方根误差为0.060 m3m-3,相关系数为0.66,使用STR代替LST进行大面积土壤湿度预测具有可行性,土壤水分的空间分布与实际情况基本一致,能够满足一般的应用需求。
Soil moisture plays an important role in the survival of animals and surface plants, the energy and material cycle between atmosphere and surface. Meanwhile, the large-scale monitoring of soil moisture is a critical indicator for water cycle, climate change, agricultural monitoring, ecological environment, geological disasters, fire and other applications. However, because of the influence of soil types, soil structure conditions, terrain characteristics, vegetation environment and human activities, the distribution of soil moisture has spatial heterogeneity characteristic, so it is still hard to find an optimal method to monitor the distribution of soil moisture in large areas (such as watershed scale). In the comprehensive experiments, the Shandian River Basin was selected as the experiment area. Vegetation Indices (VIs) retrieved from MODIS reflectance data and Land Surface Temperature (LST) were calculated as the input parameters, and then the soil moisture measured was taken as the expected output parameters. Further, the random forest model is used to calculate the feature importance of each input parameters to complete feature selection, and a soil moisture inversion model based on extreme random tree was constructed. Compared with empirical models, the soil moisture retrieval model based on extreme random tree has stronger nonlinear expression ability, and can introduce more input parameters; compared with traditional machine learning methods such as Support Vector Machine(SVM) and Neural Network(NN), extreme random tree can achieve better retrieval accuracy on small sample set by integrating several “weak learners” into “strong learners”; Compared with random forest, extreme random tree can reduce the variance and the deviation of the model to achieve better integration effects. Considering the difficulty of surface temperature measurements and the requirements of regional soil moisture monitoring, the research selected Short wave infrared Transformed Reflectance (STR) instead of LST to establish the extreme random tree model, and inversed the soil moisture map of 2°× 2° area covering experiment area of Shandian River Basin. The results show that: (1) when LST is used as the input parameter, the soil moisture retrieval model based on extreme random tree, (root mean square error 0.054 m3m-3 and correlation coefficient 0.69), has better performance than other models (support vector machine and random forest); (2) when STR is used as the input parameter, the root mean square error of prediction result is 0.060 m3m-3 and the correlation coefficient is 0.66. So it is feasible to introduce STR instead of LST to predict soil moisture in large area. Further, the spatial distribution of soil moisture is basically consistent with the actual situation, which can meet the requirements of general application. Focused on the limitation of MODIS data quality and the scale difference between single point measurement data and satellite data, the accuracy of soil moisture estimation still need to be improved. At the same time, due to the lack of long-term measured data of soil moisture, the spatiotemporal scalability of soil moisture inversion model needs to be further verified. Optical remote sensing is greatly affected by the weather, while microwave remote sensing can be the alternative choices for all-weather observation. The multi-source remote sensing data fusion should be attribute more attention to soil moisture retrieval model construction.