下载中心
优秀审稿专家
优秀论文
相关链接
摘要
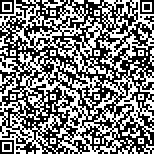
高分辨率遥感影像解译是遥感信息处理领域的研究热点之一,在遥感大数据知识挖掘与智能化分析中起着至关重要的作用,具有重要的民用和军事应用价值。传统的高分辨率遥感影像解译通常采用人工目视解译方式,费时费力且精度低。所以,如何自动、高效地实现高分辨率遥感影像解译是亟待解决的问题。近年来,随着人工智能技术的飞速发展,采用机器学习方法实现高分辨率遥感影像解译已成为主流的研究方向。本文结合高分辨率遥感影像解译的典型任务,如目标检测、场景分类、语义分割、高光谱图像分类等,系统综述了5种代表性的机器学习范式。具体来说,本文分别介绍了不同机器学习范式的定义、常用方法以及代表性应用,包括全监督学习(如支持向量机、K-最近邻、决策树、随机森林、概率图模型)、半监督学习(如纯半监督学习、直推学习、主动学习)、弱监督学习(如多示例学习)、无监督学习(如聚类、主成分分析、稀疏表达)和深度学习(如堆栈自编码机、深度信念网络、卷积神经网络、生成对抗网络)。其次,深入分析五种机器学习范式的优缺点,并总结了它们在遥感影像解译中的典型应用。最后,展望了高分辨率遥感影像解译的机器学习发展方向,如小样本学习、无监督深度学习、强化学习等。
High-resolution remote sensing image interpretation is a major topic in remote sensing information processing. It plays a vital role in the knowledge mining and intelligent analysis of remote sensing big data and has important application values in civil and military fields. The traditional methods of high-resolution remote sensing image interpretation generally use manual visual interpretation, which is time consuming and laborious and has low accuracy. Therefore, interpreting high-resolution remote sensing images automatically and efficiently is an urgent problem to be solved. The rapid development of artificial intelligence technology in recent years has made machine learning the mainstream research direction of high-resolution remote sensing image interpretation. In this study, we systematically review five kinds of representative machine learning paradigms on the basis of the typical tasks of high-resolution remote sensing image interpretation, such as object detection, scene classification, semantic segmentation, and hyperspectral image classification. Specifically, we introduce their definitions, typical methods, and applications. The representative machine learning paradigms include supervised learning (e.g., support vector machine, k-nearest neighbor, decision tree, random tree, and probabilistic graph model), semi-supervised learning (e.g., pure semi-supervised learning, transductive learning, and active learning), weakly supervised learning (e.g., multiple instance learning), unsupervised learning (e.g., clustering, principal component analysis, and sparse coding), and deep learning (e.g., stacked auto-encoder, deep belief network, convolutional neural network, and generative adversarial network). Then, we comprehensively analyze the strengths and limitations of the five kinds of machine learning paradigms and summarize their typical applications in remote sensing image interpretation. Finally, we summarize the development direction of high-resolution remote sensing image interpretation, such as few-shot learning, unsupervised deep learning, and reinforcement learning.