下载中心
优秀审稿专家
优秀论文
相关链接
摘要
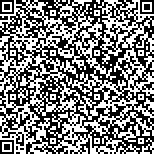
热带气旋TC(Tropical Cyclone)是影响中国的一个重要天气系统。TC强度准确估测对台风灾害防御具有至关重要的意义。本文基于第二代静止气象卫星风云四号(FY-4A)多通道扫描成像辐射计AGRI(Advanced Geosynchronous Radiation Imager)资料,建立了台风强度识别的深度卷积神经网络模型CNN(Convolutional Neural Network),对台风强度不同等级和台风中心最大风速进行了试验。结果表明,CNN模型具有良好的高维非线性处理能力和算法稳定性,能对TC强度进行有效估计,不同TC强度等级识别精度均在97%以上,近中心最大风速平均绝对误差MAE(Mean Absolute Error)为1.75 m/s,均方根误差RMSE(Root Mean Square Error)为2.04 m/s。CNN可有效挖掘卫星TC形态的深层信息,对台风强度的定量化估测具有较高的应用前景。
关键词:
遥感 热带气旋 FY-4A/AGRI卫星云图 深度卷积神经网络 强度估测A Tropical Cyclone (TC) is one of the most destructive meteorological disasters. The strong winds and heavy precipitation have significant effect on people’slives, property, and social and economic development. Therefore, the accuracy of thepath and intensity prediction of TCs is always an important consideration in meteorological research. However, considering the complexity and variability of typhoon cloud patterns, the existing objective methods are usually based on statistical linear regression.Moreover,they still have deficiencies in expressing the dynamic changes of the complex characteristics of TC cloud patterns. The deep learning algorithm performs well in high-dimensional nonlinear modelingandaccurately identifies the input mode with displacement and slight deformation.This algorithm finds significance in Tropical Cyclone (TC) monitoring with dynamic changes over time. To develop TC intensity estimation technology further in the field of satellite remote sensing, this studyapplied a new machine-learning technology to analyze and to study the TC intensity of FY-4A/AGRI data from China’s second-generation stationary meteorological satellite. First, a deep Convolution Neural Network (CNN) model was used to distinguish effectively and estimate quantitatively the TC intensity level and center wind speed. The images of day and night were placed into the convolution sampling channel of the CNN to obtain and combinesame-size spectral features. Then, multilayer convolution, pooling, nonlinear mapping, and other operations were used to mine the input characteristicsdeeply.Finally, the TC intensity was estimated. The experiment was divided into the TC intensity classification test and the quantitative estimation test of the TC center maximum wind speed. The CNN model was used to convert the recognition of the TC intensity into the pattern recognition of satellite cloud images, which could classify and identify the TC level. The experiment found that the recognition accuracy of the TC intensity was all above 95%regardless of the overall classification accuracy or the respective accuracy of day and night statistics. Compared with k-nearest neighbor, error back-propagation neural network, multiple linear regression, support vector machine, and other classical classification algorithms, itimprovesby 7-16 percentage points. Moreover, the CNN isalso superior to the classical algorithm in terms of classification accuracy. The CNN model comprises two fully connected network layers (each layer has three neurons).The TC wind speed canbequantitatively estimated by prior training samples of the network parameters. Compared with the data of Tropical Cyclone 2017 Yearbook, the mean absolute error of the wind speed was 1.75 m/s, and the root mean square error ofthewind speed was 2.04 m/s, which were lower than the corresponding errors of Deviation Angle Variance Technique (DAVT) by 85.70% and 84.38%.Thus, the CNN algorithm has a high application prospect in the quantitative estimation of typhoon intensity. As the first second-generation Chinese geostationary meteorological satellite to be launched, FY-4A has its advantages of multichannel structure and high spatial and temporal resolution. On the basis of these features, the advantages of the techniquesofthe deep neural network, and theflexible structure of CNN, this study proposesan improved CNN model thatis tailor-made for FY-4A data. The modelhas the capacity to mine the morphological characteristic of typhoons deeply and effectively and achieve high-precision typhoon intensity estimation.Thismodelhas positive research value and application prospect for the quantitative estimation of typhoon intensity.