下载中心
优秀审稿专家
优秀论文
相关链接
摘要
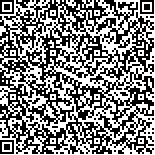
道路信息在多个应用领域中发挥着基础性的作用。光学遥感影像能够以较高的空间分辨率对目标地物进行精细化解译,可大幅增强地物目标的提取能力。充分利用光学遥感影像丰富的几何纹理信息,进行道路的精确提取,已成为当前遥感学界研究的热点与前沿问题。鉴于此,本文依据近年来大量相关文献,对现有的理论与方法进行了归类与总结,通过分析不同方法采用的道路特征组合,将道路提取方法划分为模板匹配、知识驱动、面向对象和深度学习4类方法,简要介绍了道路提取普适性的评价指标并对部分方法进行了分析与评价;最后对现有光学遥感影像道路提取的发展提出了建议和展望。
Road extraction from remote sensing image has important practical value. It presents a major issue in the field of remote sensing. In recent years, the geometric texture of the target objects of aviation and satellite optical images has been refined in the wake of the rapid development of aviation technology. Thus, this technology provides a sufficient basis for automatic extraction of road information. However, full automation of road extraction through existing methods is difficult. In view of this issue, this study collects and synthesizes the existing methods on the basis of a large number of related literature in recent years. Ultimately, these road extraction methods are divided into four categories: template matching, knowledge-driven, object-oriented, and deep learning. Template matching methods can be generally divided into rule template and variable template according to template type. The difference between the two types is whether the template can be drawn with regular graphics. Template matching mainly consists of the following three steps: template design, measure analysis, and location update. The template design can usually be set manually or by certain rules. Then, the target template is given in the measure analysis. Furthermore, the extreme value of the region is found by the measure function within the defined area. Lastly, the location of the road centerline is dynamically updated. Roads, as artificial ruled features, provide large information. Hence, knowledge-driven methods based on relevant knowledge are used in road extraction work. On the basis of the relationship between knowledge and road, this study divides the knowledge-driven methods by three kinds: geometric knowledge, context knowledge, and auxiliary knowledge. Three methods are described as follows: (1) Geometric knowledge. The model is mainly constructed on the basis of the geometric features of the road. (2) Contextual knowledge. The method utilizes road-related auxiliary knowledge (motor vehicles, trees, zebra crossings, et al.) to assist in identifying the road. (3) Assisting knowledge. Road extraction is guided by using multisource remote sensing data, vector data, GPS data, navigation data, and public source data. With the rapid increase of spatial resolution of optical remote sensing images, object-oriented methods have gradually become one of key methods in road extraction. First, the method selects the spectral and geometric feature criteria to segment image. Second, the geometric radiation similarity criterionis used to classify the segmentation region. Finally, the road extraction results are released by post-processing methods, such as mathematical morphology, matching tracking, and tensor voting. Road extraction research belongs to the problem of remote sensing image interpretation, whereas deep learning supplies a new opportunity for satellite image interpretation in a semantic direction. Deep learning extracts road information with high precision through convolution, pooling, and training. Nevertheless, road breakage and mistaken extraction problems still exist. At the end of the study, the direction of development of road extraction is envisioned. Two main trends are proposed: (1) a multimethod complementary road extraction system and (2) deep integration of deep learning and traditional methods. Thus, the detection area should be studied further.