下载中心
优秀审稿专家
优秀论文
相关链接
摘要
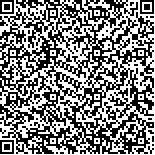
高分辨率遥感图像去噪对于提高后续图像分析、识别等问题的准确性具有重要意义。目前的去噪算法普遍存在去噪结果边缘信息模糊、易产生视觉伪影导致遥感信息丢失的缺点,针对以上问题本文提出了一个基于边缘增强的残差编解码去噪网络用于高分辨率遥感影像去噪。该方法首先将噪声图片通过低通滤波器分解成高频层和低频层,然后将含噪声信息的高频层输入到带残差模块的编解码网络中,通过采样运算在多尺度空间上学习残差映射生成残差图像,最后使用跳跃连接得到完整的去噪结果。其损失函数由逐像素和感知损失两部分组成,逐像素损失使用传统的均方根误差学习像素级信息,感知损失学习语义特征上的差异可以保留更多边缘信息,最终得到更清晰的结果,其中感知损失是由级联在后的语义分割网络提取的特征图定义的。本文对不同测试数据做去噪实验并与几个经典方法对比证明本文模型的去噪结果优于其他方法,不仅提高图像的峰值信噪比,得到最高的平均梯度值,还在视觉上取得了最清晰的结果。实验结果表明,本文提出的基于边缘增强的深层编解码卷积网络在去噪的同时可以改善边缘细节被模糊的问题,保留更多遥感地物信息,提高图像视觉效果。
Remote sensing images are often affected by noise in the process of digitization and transmission processes. Denoising is an indispensable way of improving image quality. Despite showing an excellent noise removal performance, the existing denoising algorithms however typically suffer from a common drawback. Specifically, in the learning process, some edge information is lost, thereby over-smoothing the denoising result. Given the importance of details—including sharp edges and texture information—in remote sensing images, we propose a residual encoder–decoder denoising network with joint loss (REDJ) for GF-2 satellite data. Inspired by U-net, we use a deep convolutional framework is used to learn the end-to-end mapping from noisy images to the original ones. The encoder acts as a feature extractor that captures semantic information of image contents while eliminating noise, whereas the decoder recovers the image details. The high-resolution features from the encoder are combined with the up-sampled output by skip connection. We also introduce high-frequency decomposition and residual mapping to simplify the training process by reducing the solution space. As for the loss function, we modify the traditional denoising per-pixel loss. Given a well-trained convolutional neural network for defining perceptual loss, we instead to learn the perceptual differences of the extracted features instead of merely matching the low-level pixel information. Unlike the loss of detail resulting from normal per-pixel MSE loss, we recommend a new joint loss that combines the advantages of both per-pixel reconstruction and feature reconstruction, preserves additional edge and texture information, and generates clear denoised results. We employ the GF-2 satellite images in the experiments. To obtain enough training and testing data, we divide the entire high-resolution image is divided into 1200 pictures of size 512 and then allocate 70% of these images for training and the other 30% for testing. We generate the noisy images by adding Gaussian noise. To verify the effectiveness of our proposed network, we compare our quantitative and qualitative results with those of other state-of-the-art methods, including wavelet threshold, total variation, and K-SVD. Our proposed method REDJ can obtain the best index values both of PSNR and average gradient. In the qualitative visual sense, REDJ obtains clear denoising results because of the joint of perceptual loss. Compared with other methods that produce blurred regions generated by other methods, REDJ preserves more edge information and texture details. We also compare the run times of different methods for denoising images and find that REDJ has a relatively high CPU speed and achieves an excellent computational efficiency on GPU time. This paper successfully applies deep learning theory for denoising remote sensing images. We use the proposed network is used to remove noise from high-resolution GF-2 remote sensing images and to preserve the edge contours and fine details, which is conducive to facilitate later detection, classification, and other remote sensing applications. In our future work, we will explore to handle other types of noises, especially the complex real-world noises, and consider a single comprehensive network for more image restoration tasks.