下载中心
优秀审稿专家
优秀论文
相关链接
摘要
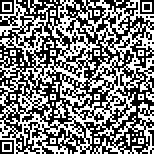
为了充分利用遥感图像中丰富的细节信息和上下文信息,提高图像语义分割精度,提出一种深度融合网结合条件随机场模型的遥感图像语义分割方法。方法在全卷积神经网络框架中增加反卷积融合结构,搭建深度融合DFN(Deep Fusion Networks)网络,通过深层网络自动获取多尺度特征,避免人工设计和选择特征,提高模型的泛化能力;同时借助反卷积融合结构,利用多尺度信息,将浅层细节信息和深层语义信息相融合,提高模型的处理精度。由全连接条件随机场引入空间上下文信息,更好地定位边界,得到最终的语义分割结果。在遥感图像数据集上的实验结果显示:(1)随着不同尺度细节信息的融入,结果的边缘轮廓越精确、接近标签图像;(2)增加了空间上下文信息后,语义分割结果边缘更细化、准确,精度更高。实验表明,该方法可以有效提高遥感图像语义分割的精度,改善结果的过平滑现象。
Image semantic segmentation refers to segmenting an image into several groups of pixel regions with different specific semantic meanings and identifying the categories of each region. In recent years, the common semantic segmentation methods that are based on Convolutional Neural Networks (CNN) have realised the pixel-to-pixel image semantic segmentation. They can avoid the problems of artificial design and selection of features in traditional image semantic segmentation methods. As a result of the pooling operation and lack of context information, the detailed information of images is neglected, the precision of the final image semantic segmentation result is low and the segmentation edge is inaccurate. Therefore, this study proposes a semantic segmentation method for remote sensing image on the basis of Deep Fusion Networks (DFN) combined with a conditional random field model.The method initially builds a DFN model in a Fully Convolutional Network (FCN) framework with a deconvolutional fusion structure. On the one hand, the multiscale features can be extracted through the deep networks, which can avoid the artificial design and selection of features to improve the generalisation ability of the model. On the other hand, the multiscale information is used in the model with the help of the deconvolutional fusion structure. The processing accuracy of the model is also improved by fusing the shallow detail information and deep semantic information. Fundamentally, the fully connected conditional random field is introduced to supplement the spatial context information towards precisely locating the boundary and obtaining final semantic segmentation results.(1)With the increase in the depth of the fusion layer, detailed information becomes abundant, the semantic segmentation results become refined and the edge contour becomes close to the label image;(2) The fully connected conditional random field model synthesises the global and local information of the remote sensing image and further improves the efficiency and accuracy of the final semantic segmentation results.From this study, we can draw the followingconclusions