下载中心
优秀审稿专家
优秀论文
相关链接
摘要
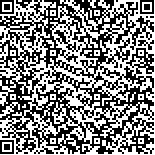
为探究具有单中波红外通道的高分四号卫星(GF-4)PMI数据在林火监测中的应用方法,通过对覆盖近年发生森林火灾的多景GF-4 PMI影像分析,采用“劈窗法”构建GF-4 PMI数据的着火点自适应阈值检测算法;在云南省玉龙纳西族自治县、俄罗斯阿穆尔州和俄罗斯外贝加尔边疆区等3个实验区进行了着火点检测应用,并以目视解译的着火点结果为参照资料,对该算法的着火点检测精度进行了评价。结果表明,该算法在这3个实验区的着火点检测准确率均高于80.0%,基于着火点检测精度验证设定的综合评价指标高于0.780,可应用于GF-4 PMI影像着火点的检测。
关键词:
高分四号(GF-4) 劈窗法 自适应方法 着火点 阈值检测GF-4 is China's first geostationary orbit optical remote sensing satellite with high-resolution ground observation. Compared with other meteorological satellites, it has a single medium-wave infrared channel with the characteristics of high spatial and temporal resolution. To explore the application of GF-4 panchromatic multispectral and medium wave infrared (PMI) in forest fire detection and provide a new method for forest fire monitoring in China.Yulong autonomous county of Yunnan province, the Amur region and the outer Baikal frontier of Russia were selected as experimental areas. During the forest fires in the experimental areas in 2017 and 2018, 12 scene images of GF-4 PMI were obtained, of which 8 scene images were used as the experimental group, and 4 scene images were used as the verification group. Statistical analysis was performed on the typical characteristics of the experimental group images, and the ‘split window method’ was used to construct an adaptive threshold detection algorithm, then the detection algorithm was used to detect the images of the verification group and compared with the results by visually interpreted.The results showed that the detection of forest fire points in Yulong autonomous county of Yunnan province, the accuracy of the algorithm in this paper was 80.0%, the omission detection rate was 20.0%, and the comprehensive evaluation index, which was based on the verification of fire detection, was 0.781. The detection of forest fire points in the outer Baikal frontier of Russia, the accuracy of the algorithm detection was 99.1%, the omission detection rate was 24.3%, and the comprehensive evaluation index was 0.858. The forest fire of 2017 in the Amur region, the accuracy of the algorithm detection was 97.7%, the omission detection rate was 22.2%, and the comprehensive evaluation index was 0.866. The forest fire of 2018 in the Amur region, the accuracy of the algorithm detection was 92.4%, the omission detection rate was 14.5%, and the comprehensive evaluation index was 0.889.The accuracy of the fire points detection in these three experimental areas was higher than 80.0%, the comprehensive evaluation index set based on the accuracy verification of the fire point detection was higher than 0.780. This algorithm could realize the fire point detection of GF-4 PMI images, and the algorithm had a higher accuracy rate of fire point detection in a large range of forest fire, but the omission detection rate of the algorithm was high and needs to be further optimized. The experimental results showed that the proposed algorithm was reliable, which could provide a method reference for forest fire monitoring in China.