下载中心
优秀审稿专家
优秀论文
相关链接
摘要
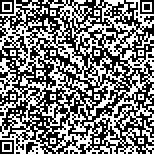
遥感影像多尺度分割是面向对象影像分析方法(OBIA)的关键步骤,分割质量直接影响OBIA的分类精度,目前多尺度分割方法都很难让分割结果同时达到全局和局部最优。本文针对上述问题,提出一种新的顾及全局和局部最优的高分辨率遥感影像多尺度分割优化算法。该算法主要包括:(1)采用局部方差准则获得多尺度分割的全局最优分割尺度。(2)对全局最优分割尺度中的过分割和欠分割对象进行优化处理,获得局部最优分割结果。(3)将局部最优化分割结果与全局最优分割结果进行融合,获得最终的多尺度优化分割结果。本文采用2个QuickBird遥感影像进行实验,验证该算法的有效性,并对实验结果进行定性和定量分析,结果表明:(1)从视觉效果来看,优化后的分割结果具有更准确的分割边界,大尺度的地物保持较好的区域性,小尺度的地物保持了更多细节。(2)从定量评价指标(RR、RI和ARI)分析:在实验1中,该算法比全局最优分割尺度的RR\RI\ARI分别提高了2.1%,2.4%,30.2%,比基于K均值优化算法分别提高了8.3%,0.1%,8.1%,比融合边界优化算法分别提高了0.7%,0.4%,17.6%;在实验2中,该算法比全局最优分割尺度的RR\RI\ARI分别提高了4.5%,2.7%,29.3%,比基于K均值优化算法分别提高了17%,0.8%,8.4%,比融合边界优化算法分别提高了1.7%,2.5%,17.2%。(3)相对典型分割算法,该算法的优化结果达到了局部和全局最优;相对其他多尺度分割优化算法,该算法同时减少了欠分割和过分割对象。
With the significant improving for the spatial resolution of remote sensing imagery, the limitation of the traditional pixel-based methods for medium and low resolution remote sensing image have become obvious. In recent decades, the Object Based Image Analysis (OBIA) has become the most popular information extraction method for the high spatial resolution remote sensing imagery. The object is the based processing units in the OBIA method, so that the segmentation method obtaining the objects is a key step in the OBIA, because the classification accuracy is directly affected by the quality of segmentation results. However, the objects in high-resolution remote sensing images show multi-scale characteristics, and it is difficult to accurately obtain the optimal segmentation results using the single segmentation scale, so that he multi-scale segmentation method have become an inevitable choice. But the multi-scale segmentation algorithms proposed in previous literatures are difficult to achieve global and local optimization. In this paper, a new multiscale segmentation optimized algorithm was proposed. The algorithm mainly includes following steps: (1) the global optimal scale in multiscale segmentation was obtained for using the local variance criterion; (2) the over-segmentation and under-segmentation objects in global optimized scale were respectively optimized to obtain the local optimization results; (3) the global and local optimized results were fused to obtain the finishing optimized results. In this paper, the two high spatial resolution remote sensing images which respectively located in Dongguan, China and Florida, USA were used to verify the effectiveness of the proposed algorithm, and the experimental results were analyzed by the qualitative and quantitative evaluation .The results were shown following as: (1) From the perspective of visual effects, the more accurate segmentation boundary were obtained in the optimized results, and the objects (such as road, farmland, and water) in the large scale maintain better regional feature, and the objects (such as tree, house, shadow)in the small scale have more detail information. (2) From the perspective of quantitative analysis by the evaluation indicators(RR, RI and ARI), the presented algorithm increased the RR, RI and ARI by 2.1%, 2.4 and 30.2% in comparison with the global optimized segmentation scale, and by 8.3%,0.1% and 8.1% in comparison with the k-means optimized method, and by 0.7%,0.4% and 17.6% in comparison with the fused boundary optimized method in the test 1, and increased the RR,RI and ARI by 4.5%,2.7% and 29.3% in comparison with the global optimized segmentation scale, and by 17%,0.8% and 8.4% in comparison with the k-means optimized method, and by 1.7%,2.5% and 17.2% in comparison with the fused boundary optimized method in the test 2. In summary, compared with classical segmentation algorithms, the proposed algorithm obtained the best segmentation results by both local and global optimization, and reduced over-segmentation and under-segmentation objects in the segmentation results. Meanwhile, the heterogeneity of objects is different in the different types of scenes, for example, the objects in the city scenes are high heterogeneity, but the objects of the rural scenes are high homogeneity. So that, the optimal segmentation parameters in multi-scale segmentation is difficult to other scenes.