下载中心
优秀审稿专家
优秀论文
相关链接
摘要
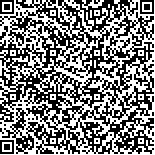
地上生物量能够有效反映作物的生长状态,其信息的实时估算对产量预测和农田生产管理都有重要意义。作物生长模型因其详尽的生理生化基础和对生长过程数字化描述能力,成为生物量估算的理想模型。近年来,研究人员利用数据同化算法将时间序列遥感数据同化到作物生长模型中,实现了作物模型由基于气象站的点模拟到区域尺度面模拟的外推,使生物量模拟结果同时具备大范围和机理性两个方面的特点。这一模式下,时间序列的遥感数据质量将对生物量模拟精度产生直接影响,作物生长后期受到光谱饱和的影响,遥感数据的作物冠层信息获取能力会出现明显下降,因此有必要对该阶段遥感数据和作物模型的结合方式进行优化,提升生物量模拟精度。本文针对东北地区春玉米生物量遥感估算存在的问题,提出了利用WOFOST作物模型结合无人机(UAV)遥感数据实现作物生长后期生物量准确估算的新思路。新思路首先利用多光谱遥感数据获取WOFOST模型具备较高空间异质性的土壤速效养分参数以提升模型的空间信息模拟能力,使其能在一定程度上摆脱点尺度模拟的限制。同时,结合集合卡尔曼滤波算法将生长前期无人机(UAV)遥感数据同化到模型中,以缩短模型单独运行时间,减少模型运行过程中的参数误差累积,实现无遥感数据参与下的短期作物生长模拟,并输出生长后期相应的生物量模拟结果。最后,本文利用地面实测数据对新方法的生物量模拟精度进行了评价。结果表明,与全生育期数据同化相比,新方法的生物量估算精度有了明显的提升(全生育期同化:R2 = 0.45,RMSE = 4254.30 kg/ha;新方法:R2= 0.86,RMSE = 2216.79 kg/ha)。
Aboveground biomass is one of the most important indicators of crop growth, and timely and accurate information on biomass is critical for crop growth assessment, yield forecast, and farm management. Crop models based on detailed physiological and biochemical information can provide digital descriptions for key vegetation processes and are ideal choices for crop biomass estimation. Given that crop models are generally designed to simulate crop growth at point scale, applying crop models to the regional scale requires knowledge of a large amount of input parameters with high calibration cost. In recent years, researchers have combined Remote Sensing (RS) and crop models by using assimilation methods to improve the ability of regional biomass estimation. However, considering that RS data provide most spatial information during model execution, any errors in these data will influence the biomass estimation accuracy.Under the influence of reflectance saturation, the crop canopy information capture ability for the RS method in the late crop growth stage is lower and errors can be hardly be avoided. In this study, a new combination method for RS data and crop model was proposed to address the saturation issue during late-stage biomass estimation. In the new method, the whole crop growth season was divided into early stage and late stage. At the early stage, the World Food Studies (WOFOST) crop model was adopted to simulate daily crop growth at point scale. Next, the Leaf Area Index (LAI) based on the time-series multispectral RS was assimilated into the WOFOST model to extend model simulation from point scale to regional scale. The RS-based LAI was calculated through a statistical model using multispectral RS data acquired by an Unmanned Aerial Vehicle (UAV). The statistical model was built according to field LAI and UAV based vegetation indices. At the end of the early stage, the key model status parameters, including dry matter of different organs, soil water, and accumulated temperature, were simulated and output by the WOFOST model.Meanwhile, soil-available nitrogen content was also estimated and transformed to the beginning of the late growth stage. At the late stage, the RS data assimilation was halted to avoid unnecessary errors caused by reflectance saturation. The estimated soil-available nitrogen and model status parameters were input to the WOFOST model, and the aboveground biomass was estimated based on the crop growth simulations. Given that the WOFOST model was only used for a short-term crop growth simulation without RS data, the error accumulation could be effectively avoided. In this study, the spring maize plots in Hongxing Farm, northeast China, were selected to apply the proposed new method and the biomass was estimated in 2015. As a comparison, the RS-based LAI for the late stage was also assimilated into the WOFOST model to estimate the spring maize biomass for the same plots. Using the field campaign data, R2 and RMSE were calculated to analyze the estimation accuracies of the two methods. Analysis results show that the accuracy of the new method (R2 = 0.86, RMSE = 2216.79 kg/ha) was higher than that of the method with RS data assimilation for the whole crop growth season (R2 = 0.45, RMSE = 4254.30 kg/ha). In conclusion, the new method can provide reliable biomass results for spring maize in the late stage by taking advantage of the WOFOST mode and UAV data.