下载中心
优秀审稿专家
优秀论文
相关链接
摘要
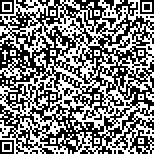
目前,估算高分辨率叶面积指数LAI(Leaf Area Index)的常用方法是采用大量地面测量数据和遥感数据建立统计模型,再用统计模型估算LAI。然而,与农田地面测量实验相比,森林地面测量实验获取的观测数据更加有限,这使得基于统计模型的森林高分辨率LAI的估算精度低,难以满足应用需求。为此,本文提出一种基于森林模型参数先验知识、使用森林研究区少量的LAI地面测量数据和归一化植被指数NDVI数据估算森林高分辨率LAI的方法。首先,获取全球20个森林实验区的LAI地面测量数据和NDVI数据,建立LAI-NDVI统计模型并提取森林模型参数的先验知识。然后,以一个新的森林站点Concepción作为研究区,将该研究区的数据分为建模数据和验证数据两个部分。使用研究区有限的建模数据对森林模型参数先验知识进行本地化校正得到优化模型,优化模型用于估算森林高分辨率LAI,使用验证数据评价LAI的估算精度。同时,选取了Camerons站点、Gnangara站点、Hirsikangas站点评价本文方法的LAI估算精度。使用地面测量LAI验证基于森林模型参数先验知识估算高分辨率LAI的结果精度,经验证4个森林站点的均方根误差分别为0.6680,0.4449,0.2863,0.5755。研究结果表明:在仅有少量观测数据时,采用本方法能有效地提高森林高分辨率LAI的估算精度。因此,本方法可为森林高分辨率LAI的遥感估算提供参考。
At present, the high-resolution Leaf Area Index (LAI) is usually estimated by statistical models, which is established by a large quantity of Vegetation Index (VI) data and ground LAI measurements. Compared with a cropland field campaign, the ground LAI measurements of the forest field campaign are less. The accuracy of the high-resolution LAI estimated by statistical models in the forest is low, and it is difficult to meet the application requirements. In this paper, a method was developed to estimate the high-resolution LAI in the forest based on the prior knowledge of modeling parameters for the forest, a small amount of forest ground LAI measurements and the Normalized Differential Vegetation Index (NDVI) data. First, for the power model which contains parameter a and parameter b, the prior knowledge of modeling parameters in the forest was achieved. 20 forest sites with a large amount of ground LAI measurements were collected. LAI and NDVI data were obtained from the 20 forest sites respectively. The LAI-NDVI statistical model which is suitable for each forest site was established with the obtained LAI and NDVI data respectively too. The values of the parameter a were extracted from the 20 statistical models, and the mean value and the standard deviation of the values were calculated to determine the prior distribution of the parameter a. The mean value of the parameter a was chosen as the prior initial value and two times of the standard deviation of parameter a was chosen as the uncertainties of the prior initial value. The same method was used to extract the prior initial value and the uncertainties of the prior initial value for the parameter b. So far, the prior knowledge of the modeling parameters for the forest was extracted. Second, the optimized LAI-NDVI statistical model was constructed for the study area. A new forest site, Concepción, was selected as the study area. The data of this site were divided into two parts: the modeling data and the validation data. The limited modeling data were used to adjust the prior initial value under the limitation of the uncertainties of the prior initial value and obtain an optimization model which is suitable for this new forest site by the optimization method SCE-UA. At last, the high-resolution forest LAIs were estimated and validated in the Concepción site. The high-resolution forest LAIs were estimated using the optimization model and the NDVIs in the validation data. The estimated high-resolution forest LAIs were evaluated by the ground LAI in the validation data. Moreover, the Camerons site, Gnangara site, and Hirsikangas site were selected as the study area to evaluate this method too. Compared the estimated high-resolution forest LAI with the ground LAI, the root mean square errors were 0.6680, 0.4449, 0.2863 and 0.5755 respectively. These results indicated that when only a small amount of ground LAI measurements is available, this method based on the forest prior knowledge could improve the accuracy of the high-resolution LAI estimation in the forest. Therefore, the method based on the forest prior knowledge of modeling parameters provided a reference for high-resolution forest LAI estimation.