下载中心
优秀审稿专家
优秀论文
相关链接
摘要
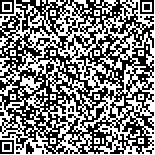
以江西省吉安市为研究区, 将5种全球地表覆盖分类数据(包括美国地质调查局(USGS)、马里兰大学(UMD)和波士顿大学(BU)生成的3套数据和欧洲生成的2套数据)以及由TM影像生成的区域地表覆盖分类数据, 分别与MODIS 1 km反射率资料结合, 利用基于4尺度几何光学模型的LAI反演方法生成研究区的LAI。在1 km和4 km两种尺度上将反演的LAI与TM资料生成的LAI进行比较, 评价地表覆盖分类数据对LAI反演结果的影响。结果表明, TM和欧洲太空局的GLOBCOVER地表覆盖分类数据用于反演LAI的结果较好, 在1 km尺度上, 反演的LAI与统计模型估算的TM LAI相关的R2分别为0.44和0.40, 在4 km 尺度上的R2分别为0.57和0.54; 其次为波士顿大学的MODIS地表覆盖分类数据, 据其反演的LAI与TM LAI相关的R2在1km 和4 km尺度上分别为0.38和0.51; 而马里兰大学的UMD和欧洲的GLC2000地表覆盖分类数据会导致反演的LAI存在较大误差, 据其反演的LAI与TM LAI 之间的一致性较差, 在1 km 和4 km两种尺度上平均偏低20%左右; LAI的反演结果对聚集度系数具有强的敏感性。该研究表明, 为了提高区域/全球LAI反演精度, 需要有高质量的地表覆盖分类数据。
In this study, six different land cover datasets were employed in conjunction with MODIS 1km reflectance data to inverse LAI of forests using an algorithm based on the 4-scale geometrical optical model in Jian City, Jiangxi Province, China. Land cover datasets used in this study include five global land cover datasets (Three were produced by the United States Geological Survey (USGS), University of Maryland (UMD), and Boston University (BU), respectively. Two were constructed in Europe.) and a regional land cover map produced using Landsat TM images. For assessing the impact of land cover on the inversion of LAI, LAI images inversely produced with different land cover datasets were compared with LAI data sampled from a 30 m LAI map at 1 km and 4 km scales, respectively. The 30 m LAI map was produced with TM reflectance images and ground measurements of LAI. The results show that the land cover datasets of TM and GLOBCOVER which was created by European Space Agency are the best for the inversion of LAI in this study area. At 1 km scale, the R2 values of LAI inversed using TM and GLOBCOVER land cover datasets with TM LAI estimated using an statistical model are 0.44 and 0.40, respectively. At 4 km scale, these R2 values increase to 0.57 and 0.54. The MODIS land cover data of BU is the third best data for the inversion of LAI, the R2 values between LAI inversed using this land cover dataset and TM LAI are 0.38 and 0.51 at 1 km and 4 km scales, re-spectively. The land cover datasets of UMD and European GLC2000 resulted in large discrepancies between inversed LAI and TM LAI. The averages of LAI inversed using these two land cover datasets are about 20% lower than TM LAI at 1 km and 4 km scales. Sensitivity analysis shows that inversed LAI is sensitive to clumping index. This study proved that reliable land cover data is required for improving the accuracy of inversed LAI at regional/global scales.