下载中心
优秀审稿专家
优秀论文
相关链接
摘要
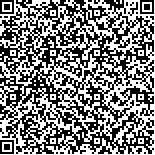
以提高冬小麦种植面积估算精度为目标, 选取种植结构复杂的都市农业区, 采用QuickBird影像数字化农田地块边界, 以多时相TM影像为核心数据源, 以地块为基本分类单元, 进行不同特征向量组合、不同分类器的冬小麦地块分类方法研究, 并对比分析了基于地块分类和基于像元分类的冬小麦种植面积估算精度。研究结果表明, 基于地块分类的冬小麦种植面积估算方法的总量精度和位置精度均高于像元分类; 植被指数和纹理信息的引入有助于进一步提高地块分类精度; 支持向量机与最大似然均能得到高达97%的总量精度和90%的位置精度, 支持向量机地块分类所需的训练样本量远低于最大似然, 因此支持向量机更加适合于冬小麦地块分类; 冬小麦错分与漏分情况大多发生在细碎地块, 其面积总量较小, 而大地块错分和漏分较少, 因此相对于像元分类, 地块分类能在整个区域能得到较高的冬小麦位置精度和总量精度。
With the significantly improved data availability in remote sensing technology, mid-resolution images have become the primary data source for crop sown area estimation in large scale. However, it is still difficult to solve the problems of spectrum heterogeneity in one field and spectra similarity between fields, especially in transitional region by using mid-resolution images. In order to maximally avoid above motioned problems and accurately measure the sown area of winter wheat, this paper developed per-field classification method and tested the method in an urban agriculture region with complex planting structure through several steps: first, digitalizing field boundary from QuickBird image; second, extracting characteristic index including spectrum and texture information as well as vegetation index for each field from the multi-temporal TM images; third, operating support vector machine (SVM) and maximum likelihood classification (MLC) with different field characteristic index; finally, estimating the accuracy of our method. Results show that the per-field classification method has a higher accuracy than per-pixel classification both in amount (estimated sown area of winter wheat divide by reference sown area of winter wheat, Kr) and position (equal to product accuracy, Kp). Although both SVM and MLC could get very high amount and position accuracy (97% and 90% respectively), the estimations of SVM are more stable. The errors of per-field classification mainly happened at the fragmentized parcels. Additionally, characteristic information could enhance the performance of per-field classification. Our method also has an outstanding advantage that no optimum period requires on satellite imagery which could enhance practicability and operationality of our method.