下载中心
优秀审稿专家
优秀论文
相关链接
摘要
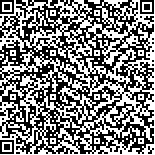
建立了基于支持向量机的遥感水质参数反演模型, 构建了基于浮点数编码的遗传算法优选模型参数。以渭河为研究对象, 基于高分辨率多光谱遥感SPOT-5数据和水质实地监测数据, 分别建立了一元和多元经验模型进行渭河水质参数的反演。在样本数目有限的情况下, 提出的GA-SVM方法的反演结果比神经网络和传统的统计回归方法好, 且各方法的多元回归结果均好于一元回归的结果。SVM具有强的非线性映射能力, 适合小样本情况, 由GA实现了模型参数的自动优选, 使GA-SVM用于解决回归问题表现出优势。将机器学习和全局优化智能计算方法引入, GA-SVM为渭河陕西段的水环境遥感监测提供了一种新方法, 取得了较好的反演结果。
This paper establishes the retrieving models of water quality parameters by remote sensing based on support vector machine (SVM), and proposes a self-adaptive optimization algorithm for the selection of SVM model parameters using genetic algorithm (GA). Using high resolution multispectral SPOT-5 data and in situ measurements, we construct univariate and multivariate empirical models for retrieving water quality parameters of Weihe River in Shaanxi province. The capability of the proposed GA-SVM method is obviously better than the neuron networks and the traditional statistical regression methods even for limited samples. And the results of multivariate models are always better than that of univariate models for these methods. Since SVM has the ability of non-linear mapping, fitting for small samples, and the model parameters are selected automatically by GA, GA-SVM method shows distinct superiority in solving our problems. By introducing the new method of machine learning and intelligent computing method for global optimization, GA-SVM provides a new approach for water quality monitoring by remote sensing, and can obtain better results for Weihe River in Shaanxi.