下载中心
优秀审稿专家
优秀论文
相关链接
摘要
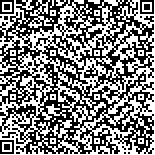
遥感时间序列数据(MODIS,NOAA/AVHRR,SPOT/VEGETATION等)在植被生长监测、物候信息提取、土地利用类型监测等诸多领域得到了广泛应用,是生产研究的重要数据源之一.由于传感器、云层大气等影响,遥感时间序列数据存在着严重的噪声,应用前必须进行序列滤波重建工作.综述现有各类滤波重建方法,对研究中广为采用的3类主要方法(基于最小二乘的非对称高斯函数拟合、SavitZky-Golay滤波、基于离散傅里叶的系列分析方法)集中阐述其理论基础、应用步骤和优缺点.总结当前遥感时间序列滤波重建方法需要进一步改进之处.
Remote sensing time-series have been applied successfully in various fields, such as vegetation change monitoring,phonological(seasonality) information extraction, land use dynamic classification etc. It is one of themost importantdata sources forkinds of researchwork&engineering projec.t However, due to the effectof sensor, cloud, and atmospheric conditions, there are serious residualnoise in time-series data. Therefore, prior to furtherapplications, it is need to filter residualnoise to reconstruct the series.Manymethods have been developed to solve this problem.In this paper, the following methods are summarized firs,t including Maximum Value Composite (MVC), Best Index Slope ExtractionAlgorithm (BISE), TemporalW indowsOperation (TWO), AsymmetricGaussian Function Fitting Approach (AGFF),Savitzky-Golay Filtering (S-GF), Harmonic Analysis Algorithm (HAA), Local Maximum Fitting (LMF). MVC is more acceptable than othermethods because it is usefulwhen producing remote sensing time-series products.But the products are the primary ones and containmuch residualnoise. Thismethod is helpless to reconstruct the series further. In fac,t the data needed to reconstructbefore applications of theproductsmade according toMVC. BISE uses a sliding timewindow to capture localmaxima. It requires the determination of the sliding period and a threshold foracceptable percentage increase. TWO can reduce the noise caused by cloud and atmospherewithoutauxiliary data.However,the requirementof the parameters from experience limits its application.AGFF and S-GF are two strategies developed in recentyears.LMF,comparedwithHAA, firstfiltersnoise and then reconstructs the data processed.Then threemost frequently-used approaches,AGFF,S-GF and HAA, are introduced in detail in terms of the basic theories,application steps and advantages & disadvantages. AGFF employsmore than two combinativeGauss-shape curves to fit the series.Every combination simulates a cycle of plant life. Finally, these combinative fitting curve are joined together to represent the reconstructed data. By this strategy, every combination is independen.t AGFF which ismore flexible than othermethods could avoid curve distortion. But the parameter, the runningwindow used to find a consistent setofmaxima andminima, is difficult to retrieve.Thus itmakes the furtherprocess less reliable and steadier. S-GF is a process of smoothing and filtering in essence. Two parameters control the result one is the scale of the smoothing window, and the other is convoluting integer. The two parameters are all obtained depending on experience. Meanwhile,a new variable, fitting-effect index is introduced to control the iteration stop. It is more advanced than the other methods which terminate the iteration by a given threshold. S-GF has receivedmore concern because its product can clearly reflect long time trendy and localchange information. Meanwhile its products are less subject to special scale and remote sensor. HAA is a term standing foramethods setwhich useharmonic to fitdatawhile simulate the seasonalregulation. These methodsmake use of sine or cosine to fit the data and simulate the seasonal regulationwhich ismuch concerned. Twomainmethods ofHAA are Seller Algorithm and HANTS.At las,t some comments are given, discussing thedefectsof the approaches, andwhich aspectsneed to be improved and how to.For example, most of parameters needed in these reconstruction methods have to be decided by experience. Thus subjective errors from operatorwould affect the reliance and stability ofproducts. So new strategy or improved ones need to solve this problem.