下载中心
优秀审稿专家
优秀论文
相关链接
摘要
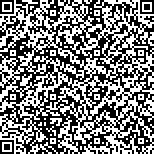
提出了基于蚁群智能的空间选址模型,通过蚁群智能和GIS的结合来解决复杂的空间优化配置问题.这种启发式的智能搜索方法大大提高了空间搜索能力.为符合选址问题的求解,从信息素更新方式和禁忌表调整策略两方面对基本蚁群算法进行改进.同时,为了使得模型能实用于大区域的基础设施选址,提出了"分步逼近"的策略,取得了较好的效果.将所提出的模型应用于广州市公共没施的空间优化选址.实验结果表明,该方法比简单搜索方法和遗传算法更有优势.
Optimal site search for sitting facilities is crucial for the effective use and management of resources and it is also a common task forurban planning. The brute-forcemethod has difficulty in solving complex site search problems especially in large scale areas. In this study, a locationmodel is proposed based on antcolony algorithms (ACO). Itcombines antcolony intelligence andGIS to solve the problems of complicated spatialoptimalallocation. ACO has strong search ability fora huge volume of spatial data.At firs,t the algorithm is modified about the strategy of pheromone update and Tabu table adjusting to fit the sites location problem. Spatialallocation problems usually have a large setof spatialdata and only a few targets. The pheromone evaporates very fastbecause the selected cells only amount to a smallpercentage ofall the cells. The positive feedback is tooweak to play a role in the optimization. Amodification is to incorporate the strategy ofneighborhood pheromone diffusion in defining pheromone updating.At the same time, an optimal resultfor sites selection usually doesnot include two nearcandidate cells together, so a restrictedTabu table updating strategy is adopted which resembles the strategy of neighborhood pheromone diffusion. Then another important modification is to adoptamulti-scale approach to alleviate the computational demand in conducting large-scale spatial search. This includes two phases ofoptimization. Firs,t a coarser resolution is used for the identification of rough locations of targets usingACO.Then the next round ofoptimization is implemented by just searching the neighborhood around these initial locations in the original resolution. This two-phase procedure ofoptimization can thus significantly reduce the computation time.The study area is located in the city ofGuangzhou. This optimization problem considers two spatial variables, population distribution and transportation conditions, which are obtained from GIS. The raster layers have a resolution of 100×100m with a size of250×250pixels. A comparison experiment is conducted among themulti-scaleACO, ACO, genetic algorithms and simple search algorithms. Experiments indicate thatthismulti-scale ACO method can produce similar resultsbutuse much lessercomputation time, comparedwith the single ACO method. Thismethod has better performance than the single searchmethod and the genetic algorithm method for solving site search problems. ACO has obtained the utility improvementof2.7%—5.5%, comparedwith the single searchmethod. ACO has slight improvementof the totalutility value over theGA method, but is able to reduce computation time significantly.Its computation time is only12.5%—29.5% and8.8%—20.3% of those of the single searchmethod and the GA method respectively.In conclusion, integratingACO withGIS is importantfor solving real-world site selection problems. The integration allows these two techniques to bemutually benefited from each other. ACO provides an efficient distributed computation algorithm while GIS provides useful spatial information. The comparison experiment indicates that the proposedmodel has better performance thanGA\nand SSA especially in the computing time for solving site search problems.