下载中心
优秀审稿专家
优秀论文
相关链接
摘要
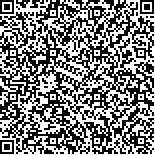
在运用混合高斯密度模型对差分影像建模的基础上,分别采用顾及上下文信息的概率松弛迭代法和马尔可夫随机场模型法进行影像的变化检测.首先,提出一种运用遗传K均值算法与EM算法联合解算高斯混合密度模型参数的方法,该方法可以自动地解算出模型的统计参数,结果与手工选择样本的解算结果完全一致.然后,比较概率松弛迭代法以及马尔可夫随机场模型法的影像变化检测效果,得出基于模拟退火法的马尔可夫随机场法效果较好的结论.最后,对传统的基于模拟退火法的马尔可夫随机场方法进行改进,提出了一种变权马尔可夫随机场方法,检测结果能更好地保持影像的结构性,并有效去除了孤立噪声.
Multi-temporal remotely sensed imagery change detection is a hot topic in recentyears. Most researchers pay attention to statisticalpattern recognition principal to solve the problem. In this paper, we discuss the problem from three aspects. Firstly wefocus onGMM model statistic coefficients resolvemethod. The ExpectationMaximization(EM) is themost commonly method to calculate GMM coefficients. However, EM algorithm often converges to local value. So we combine genetic k-means algorithm(GKA) withEM to modify i.t Using the initial clustering result obtained by GKA, we are able to initialize EM globally. The combination helpsEM search out globally optimal solution and enhances the automatic degree. When we get the global optimal results, it is easy forus to obtain change detection result using Bayes Rule forM inimum Error (BRME). However, the BRME doesn t take into account the image s contextual information. It iswellknown thatone pixel belongs to“change”or“no change”depends not only on itself, but also on its neighbor pixels. There are two ways to model contextual information. The first is probability relaxation iteration, and the second isMarkovRandom Field (MRF). MRF has two commonly used solutionmethods;one is IteratedConditionalMethod (ICM), and the other is SimulatedAnnealing (SA). In this paper, we compare the three spatial contextual change detectionmethods using visual effectand kappa coefficien.The experiment shows that MRF based on simulated annealing has betterperformance than the other two.Through the above experiments, we find that traditional MRF deals all pixels equally and ignores the neighbor local features. In fac,in different region, the spatial information has different impac.t We analyze the impact and propose variable weight MRF method.It can adaptively vary spatial impact according to different image local features. It has virtuesofpreserving structuralchange and filternoises.The experimentproves thatvariableweight MRF gets thebest resul.