下载中心
优秀审稿专家
优秀论文
相关链接
摘要
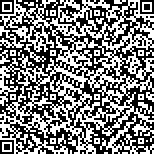
提出了一种基于误差分析的组合分类器,通过结合两种监督分类方法,提出的算法分别估计了两种监督分类方法在计算过程中的误差,给出了规则输出的置信区间,再根据置信区间的大小对两种分类方法的输出结果进行加权平均,从而得到更精确的规则输出.利用该方法对遥感图像进行分类实验,在不同训练样本分布与不同训练样本数量的情况下,比较新的组合分类器与单一分类器的精度.结果表明新的组合分类器能够取得比单一的分类器更高的分类精度.结果还显示出,两个分类器的独立性越强,组合分类器的效果越好.另外一个实验比较了新的组合分类器与和式规则组合分类器的分类精度,结果仍显示出了新方法的优越性.
Remote sensing iswidely used inmapping land use/land cover types and monitoring land use /land cover changes from regional to global scale. Supervised classificationmethod is a powerful tool in extracting land cover and land\nuse information from remotely sensed mi ages. Although many supervised classification method have been developed in machine learning field, there are not a universal best performingmethod yet. That is,different kinds of classification methods have theirown advantages and defects. Thisphenomenon is called selective superiority. It isnecessary to explore amethod thatcan integrate advantagesofdifferentclassifiers and avoid theirweakness. Combining classifier sproperlymay mi prove classification accuracy, because different classifiersmay have differentmistake sets. Combined classifiers have been studiedwidely inmachine learning field; however, itwas seldom studied in remote sensing mi age classification. This paper proposed one type of combined classifier based on error analysis, which incorporates the rule outputs ofmaxmi um likelihood classification (MLC) and supportvectormachine (SVM), to achieve higher classification accuracy.MLC is themostwidely used classificationmethod in computer processing of remotely sensed mi ages. It is based on classical statistical theory and has solid probabilitymeanings. However, the classified accuracy of thismethod would be affected seriously if the training sample distribution does not follow normal distribution. SVM is a newly developed classifier, which is based on statistical learning theory. SVM is robust for small sample, and it has shown a good performance inmany studies. However, the originalSVM classifier is a binary classifier, which needs to be extended to a multi-class classifier through extraworks.How to effectively extend binary SVM tomulti-class classification is still an ongoing research issue and itprobably affect the performance of SVM. The newmethod proposed in thispaper firstestmi ates the errorsoftwo classifiers, which are denoted by the confidence intervalsofrule outputs, then combines their rule outputs withweights depending on the confidence intervals, and finally acquires a more accurate rule output. Classification expermi entswere conducted on case study area (Summer Palace area in Beijing). Classification accuracies of the combined classifier and two single classifiers were compared with different sample distribution and different sample amount. And the results demonstrated that the new combined classifier can acquire a higher accuracy than other two classifiers. The results also revealed that combined classifier performs betterwhen two classifiers aremore independent.Another compared expermi entwas done between new combined classifier and previous combined classifier by averaging,and resultalso showed thatnewmethod had betterperformance. However, there are still some defects in the newmethod.Firstly, error analysis is not completely finished for the two classifiers; secondly, error analysis based on classical statistical theorywould be too optmi istic forMLC. Although there are some disadvantages in the new combined classifier based on error analysis, it stillhas shown promising potential in remotely sensed mi age classification.