下载中心
优秀审稿专家
优秀论文
相关链接
摘要
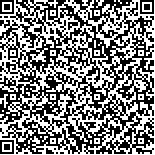
土地覆盖的短期时空变化模式研究,对土地覆盖的快速、动态监测具有重要意义,也是遥感研究的新热点。本文利用2000—2001年的时间序列Radarsat图像,采用功率谱分析方法,对土地覆盖的短期时—空变化的周期特征进行了分析,由此建立了基于时间序列影像分析的神经网络预测模型,从植被主要生长季节的时间序列雷达卫星影像获取训练样本,对研究区域的典型土地覆盖的短期动态变化过程进行了学习。学习后的模型能够利用多个时间序列的Radarsat影像对下一时刻的影像进行模拟,并进一步检测变化。在模拟结果基础上,定义相对变化距离函数和检测门限,对模拟影像及实际影像中的变化区域进行了检测。检测精度范围在66.67%(农村居民点)—91.67%(水体)之间,平均检测精度为81.66%。由于时间序列信号的引入,神经网络模型能够较好地获取土地覆盖的短期动态变化信息。
Regional dynamic monitoring is gaining rising interests in landuse/land cover study.In this article,a short-term land use/land cover change detection method was proposed,which takes periodic change of land cove into account and performs change detection between simulated image and actual image.Eight scenes of Radarsat image of Pearl River Delta was used for experiment.First,periodogram analysis was carried out on the time-series data to get the temporal pattern of the study area.Some land cover like paddy,cultivated land,orchard and forest reveal periodic variation during the research span.Thus various temporal dynamics of these land covers should be taken into account to acquire accurate short-term change detection.Then,a time-based neural networkprediction model(TNN) was built for time-series forecasting.Ten types of land cover with different temporal pattern were classified and four scenes of Radarsat images in vegetation growing seasons(April,June,August,October) in 2000 were used for network training.Land-cover type was classified based on their temporal variation.The first three scenes were used as the input and the last scene was used as the output(to be predicted).The training result showed stable and precise simulation of TNN.In the third step,the first three scenes of Radarsat images in 2001 was taken as the input to the network and the forth scene was simulated.Finally,a distance function was defined and change threshold was set for change detection.The simulated result was used to detect the change between simulated image and actual image.The detection assessment shows that neural network simulation could well represent the short-time non-linear change of land use/land cover.The detection precision ranged from 66.67%(rural residential area) to 91.67%(water).Other land cover type like paddy field(83.33%) and orchard(71.43%) also got relatively high precision,corresponding to their notable variation in time-series images.The average detection precision reached 81.66%,which is a satisfying result for our primary experiment on short time change detection.To sum up,this article testified the possibility of short-term change detection under dynamic cally changing environment.So far there is still few method applicable for short-term change detection.TNN network proposed in this article is a meaningful attempt for research in this field.