下载中心
优秀审稿专家
优秀论文
相关链接
摘要
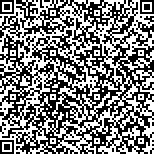
本文综述了国际遥感分类研究,使用Landsat7 ETM+遥感数据和地理辅助数据,应用BP神经网络方法,将莽汉山林场作为研究区进行了遥感影像的分类研究。比较了BP神经网络分类与最大似然、简单和复杂非监督分类法之间的类型与数量精度。BP神经网络分类的总类型精度是70.5%,总数量精度为84.65%,KAPPA系数是0.6455。结果说明BP神经网络的分类质量优于其他方法,其总的类型精度与其他三种分类方法相比分别增加了10.5%、32%和33%,总的质量精度增加了5.3%。因此,辅以地理参考数据的BP神经网络分类可以作为一种有效的分类方法。
In this paper, we present the results ofour research to evaluate the accuracy of the back propagation neural networkmethod to classify forestvegetation using a27July2001Landsat7ETM+image of the Manhanshan Forestry Center. The type and quantitative accuracy of the back propagation neuralnetwork are comparedwith themaximum like-lihood, the simple and the complex unsupervised classificationmethods. The total cover type accuracy ofback propaga-tion neural network classification is70·5%, the total quantity accuracy is84·65%, and the KAPPA coefficient is 0·6455. Our results indicate that the total type accuracy increases10·5%、32% and33% respectively compared to the other three classificationmethods. Totalquantitative accuracy increases5·3%. It is evident that the classification quality of the back propagation neuralnetwork is better than the othermethods. Therefore, the back propagation neuralnetwork is an effective and accuratemethod of classifying forestvegetation.