下载中心
优秀审稿专家
优秀论文
相关链接
摘要
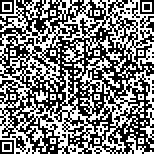
本文将遗传算法(GA)应用于非监督训练,提高了遥感数据的分类精度。遗传竞争学习算法(GA-CL)综合了遗传算法和简单的竞争学习算法,可用于改进非监督训练的结果。遗传算法在典型样本聚类的过程中可以避免得到局部最优值。Jeffries-Matusita(J-M)距离法是通过统计测量两个训练类别之间的分离度,可用于评价这种算法。将此算法应用于TM数据的结果显示,遗传算法改进了简单的竞争学习算法,与其他非监督训练算法相比,其提供了K-均值,GA-K-均值和简单的竞争学习算法。
This paper conveys the application of genetic algorithms(GA) which are used to improve unsupervised training and thereby increase the classification accuracy of remotely sensed data. The genetic competitive learning algorithm(GA-CL), an integrated approach of the GA and simple competitive learning(CL) algorithm, was developed for unsupervised training. Genetic algorithms are used to improve the training results for the algorithm. GA is used to prevent falling in the local minima during the process of cluster prototypes learning. The evaluation of the algorithm uses the Jeffries-Matusita(J-M) distance, a measure of statistical separability of pairs of trained clusters. Experiments on Landsat Thema-tic Mapper(TM) data show that the GA improves the simple competitive learning algorithm. Comparisons with other unsupervised training algorithms, the K-means, GA-K-means, and the simple competitive learning algorithm are provided.