下载中心
优秀审稿专家
优秀论文
相关链接
摘要
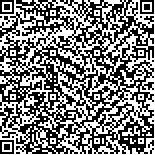
本文提出了一种新的结合多光谱和变化检测技术的多时相卫星数据集分类方法。该方法以数理统计中的最近邻法为基础,其目标函数是使得正确分类的平均概率得到最优化,即把每个分类类别看成同等重要。该新算法被应用于一个农业作物分类的研究区域,并利用覆盖该区的不同季节的SPOT和LANDSAT TM多时像影像。结果表明,与单时像影像相比,使用五个不同季节的多时像影像可以充分地提高分类精度。为了说明该方法在大尺度范围内的效果,本文选取瑞典道拉河流域作为研究区。由于不同地物的分布高度重叠,不可能得到像元水平上满意的分类精度。这就需要引进一种新的概念:像元概率分类法。基于像元的概率向量可用于判别传统分类法的可靠性并测量单个像元的不确定性(熵)。概率分类法同时提供了不同地物的面积的无偏估计,无论所感兴趣的区域的大小。这已经在不同特性的耕地试验点进行了检验。
In this paper,a new approach for classification of multitemporal satellite data sets,combining multispectral and change detection techniques is proposed.The algorithm is based on the nearest neighbor method and derived in order to optimize the average probability for correct classification,i.e.each class is equally important.The new algorithm was applied to a study area where satellite images(SPOT and Landsat TM) from different seasons were used.It showed that using five seasonal images can substantially improve the classification accuracy compared to using a single image.As a large scale application,the approach was applied to the Dal River drainage basin.As the distributions for different classes are highly overlapping it is not possible to get satisfactory accuracy at pixel level.Instead it is necessary to introduce a new concept,pixel-wise probabilistic classifiers.The pixel-wise vectors of probabilities can be used to judge how reliable a traditional classification is and to derive measures of the uncertainty(entropy) for the individual pixels.The probabilistic classifier gives also unbiased area estimates over arbitrary areas.It has been tested on two test sites of arable land with different characteristics.