下载中心
优秀审稿专家
优秀论文
相关链接
摘要
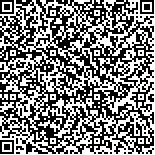
高光谱遥感能提供数十至数百个窄波段的光谱信息,从而能够依据地物的诊断性光谱特征进行地物识别。然而,高光谱遥感在提供丰富光谱信息的同时,波段间的相关性和冗余性制约着高光谱遥感的应用。因此,特征参数选择是高光谱遥感分类中最关键的环节之一。首先讨论EO-1/Hyperion的传感器特征,并对其L1R数据进行辐射校正、去条纹、Smile效应纠正等预处理工作。其次利用从图像中提取的典型地物的光谱曲线,采用光谱重建理论获得用于逼近光谱曲线的基函数及其对应的光谱区间。然后采用逐步增加光谱区间,并调整波段中心位置和宽度的方法,得到稳定的光谱区间。最后将光谱区间内的几个原始高光谱波段合成一个宽的波段,得到几个较宽波段的仿真图像,并对其进行分类。结果表明,基于光谱重建的特征参数选择方法获得的分类,总体精度高达92%,充分说明了该方法的有效性。
Developments in detector technology and microelectronics present new opportunities for remote sensing. For example, the spaceborneHyperion, flown on EO-1 satellite, acquires mi age data in 220 spectral bands over the spectral range of 0·4—2·5μm at approxmi ately 0·01μm spectral resolution with 30m spatial resolution. Those hyperspectral data can provide abundant information, and make it possible to detect diagnostical spectral characteristics, such as red-edge drift, etc.However,there is relatively high correlation between differentbands andmuch redundancy in hyperspectraldata sets. Therefore, one of themost mi portant procedures is to selectoptmi albands for extracting information from hyperspectral data effectively.In this paper,we first discuss the characteristics of EO-1/Hyperion instrument, and apply several mi portantpre-processing procedures toHyperion L1R data, such as radiometric calibration, destriping, smile correction, and geometric correction, etc.Thenwe apply spectrum reconstruction approach, which uses several basis functions and corresponding spectral intervals to describe the spectrum extracted from Hyperion hyperspectral data sets in Subei region, China. The feature selectionmethod based on spectrum reconstruction is incrementally adding bands to the initial bands, followed by adjustment of band widths and locations. At last, we can aggregate severalHyperion bands into a new smi ulated band in each intervaland applyMLC mi age classificationmethods to it.The overall accuracy can be as high as 92% compared with in situmeasurement,which supports the validity of this feature selectionmethod.