下载中心
优秀审稿专家
优秀论文
相关链接
摘要
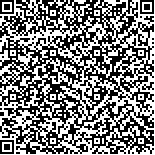
本文利用OM IS高光谱数据,研究了决策树算法(Decision Tree,DT)特征选择的特点以及特征选择对决策树分类结果的影响。设计了三种特征选择方法:SEP,MDLM和RELIEF,将它们与DT特征选择的结果以及特征选择后的分类精度(考虑了三种分类器:最大似然法、后向传播神经网络、最邻近法)进行对比,并分析了这三种特征选择方法对决策树结构和分类精度的影响。结果显示,DT是一种比较好的特征选择方法;经过特征选择后再生成的决策树比直接生成的决策树,用到更少的特征(平均减少了43.36%)、有更多的节点(平均增加了18.61%)和更高的分类精度(平均提高了0.35%),当样本数量少时,分类精度的提高幅度最大,而树的大小却基本没有增加。
In thisarticle, OMIShyperspctraldatawasused to study feature selection abilityofDT(DecisionTree) algorithm and the mi pacts of feature selection on DT. The DT was compared to three designed feature selection methods(SEP, MDLM and RELIEF) based on feature selection results and classification accuracy in which three differentmethods (ML、BPNN and 1-NN) were applied. Moreover, the mi pacts of the three designed feature selectionmethods onDT classification results atdifferent training sample sizeswere analyzed. Results indicated that DTwas a good feature selectionmethod. After feature selection, DT algorithm outputted to those classification trees thatused fewer features(average decreasewas43·36% ), had fewer tree nodes(average increasewas18·61% ), and had higher classification accuracy(average increasewas0·35% ). When the training sample sizewas smal,l accuracy miprovementwas themost significantandmeanwhile the tree size scarcely changed.