下载中心
优秀审稿专家
优秀论文
相关链接
摘要
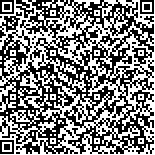
提出了基于支撑向量回归的高光谱混合像元自动分解.首先利用投影迭代的方法自动寻找到影像的典型地物光谱,然后利用Hapke近似函数模拟出非线性的训练和测试数据.支撑向量回归的混合像元分解方法与基于基函数分解方法的不同点是不需要预先确定非线性的映射形式,它通过核函数,把像元矢量从低维空间映射到高维特征空间,使得在特征空间中构造的线性光谱组合对应着原始空间(像元空间)的非线性组合特性,从而揭示了典型地物光谱之间的高阶性质,提高了混合像元的分解精度.实验结果证明,这种方法具有很高的混合像元的分解精度.利用模拟数据作分解精度的评价,表明97%以上的像元分解绝对误差不大于10%,而各类总体平均平方根误差均小于3.5%.
SpectralMixture Analysis(SMA) is a straightforward and efficient approach to the spectral\ndecomposition ofhyperspectral remotely sensed scenes, Once a SMAmodel isdeveloped, land coverproportions\ncan be estmi ated from pixel values throughmodel inversion. In this paper, we propose to estmi ate abundances\nfrom hyperspectral mi age using supportvector regression(SVR) method. SVRmethod for abundance estmi ation\ncan be essentially regarded as function approxmi ation and generalization problem. Differing from othernonlinear\nregressive approaches which require predefined nonlinear mapping functions, this method transferred each\nspectralpixel into a high-dmi ension feature space by a kernel function, whichwill result in a spectralpixel in a\nfeature space consisting of possiblymany nonlinear combinations of the spectral bands of the original spectral\nsignature. In thisway the higherorder relationshipsbetween themixed pixels are exploited in the feature space.\nProjection iterative method has been used for endmember abstraction from the mi age, and then smi ulating\nnonlinear training and testing data byHapke’s approxmi ation function. Expermi entof smi ulating data and real\nhyperspectral mi age (Pushbroom Hyperspectral Imager, PHI) are conducted to validate the procedures. The\nexpermi ents show that themethod can provide better result of abundance estmi ation for hyperspectral mi age as\ncomparedwith thatofradialbasis function-neuralnetworks. In our smi ulating test, over97% of the totalpixels\nin the mi age liewithin the bound of±0·1, and theRMSE are nomore than 3·5%.