下载中心
优秀审稿专家
优秀论文
相关链接
摘要
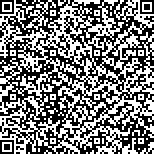
介绍了目前国际上流行的两种决策树算法———CART算法与C4·5算法,并引入了两种机器学习领域里的分类新技术———boosting和bagging技术,为探究这些决策树分类算法与新技术在遥感影像分类方面的潜力,以中国华北地区MODIS250m分辨率影像进行了土地覆盖决策树分类试验与分析。研究结果表明决策树在满足充分训练样本的条件下,相对于传统方法如最大似然法(MLC)能明显提高分类精度,而在样本量不足下决策树分类表现差于MLC;并发现在单一决策树生成中,分类回归树CART算法表现较C4·5算法具有分类精度和树结构优势,分类精度的提高取决于树结构的合理构建与剪枝处理;另外在决策树CART中引入boosting技术,能明显提高那些较难识别类别的分类准确率18·5%到25·6%。
Decision tree classification algorithms have significantpotential in remote sensing data classification. In this\nresearch, two popular decision tree algorithms———CART and C4·5 are presented, and two techniques known as boosting\nand bagging inmachine learning area are introduced. We examined thesemethods tomaxmi ize classification accuracies\nusing these decision trees and techniques tomap land cover ofHuabei area in China fromMODIS 250m data. The result\nindicates that decision tree with abundance training samples has higher classification accuracy than maxmi um likelihood\nclassifier(MLC) in the land cover classification test, whereas insufficientsamples resulted in a lower accuracy fordecision\ntree than MLC. The result also shows CART algorithm has more advantageous than C4·5 algorithm in classification\naccuracy and tree structure. And the decision tree classification accuracy depends on the optmi al structure and pruning\nprocess. We also tested the behaviour ofboosting and bagging techniques combinedwith CART and the result shows that\nadding boosting technique to decision tree can increase classification accuracies by 18·5%—25·6% for the poorly\nseparable classes inMLC.