下载中心
优秀审稿专家
优秀论文
相关链接
摘要
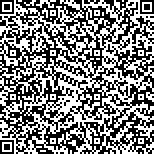
混合像元的存在是影响遥感图像分类精度的主要原因,模糊分类是进行混合像元分解的重要方法,其效果的好坏取决于各像元分类后对各类别的隶属度值能否准确地反映像元的类别组成。当非监督分类中的聚类数目与实际类别数目不符,或者监督分类中训练样本存在未训练类别时,常用的模糊c-均值(FCM)方法的效果将大大降低,而可能性c-均值(PCM)方法则可以解决这个问题。该文提出了基于PCM算法的遥感图像混合像元分解方法,并用监督分类方法实例说明PCM方法的优越性。
The existence of mixed pixels is the main factor influencing the classification accuracy of remotely sensed image. Fuzzy classification is an important method of unmixing the mixed pixels. Its results depend on how accurate the membership value to various types of each pixel after classification corresponds to its actual component. If the clustering number is not equal to the actual type number in the unsupervised classification, or there are some types untrained in the supervised classification, the accuracy of the popular algorithm, namely Fuzzy c-means (FCM) will be degraded. Fortunately, Possibilistic c-means (PCM) is insensitive to it and can work well. This paper proposes the pixel unmixing method of remotely sensed image based on PCM algorithm. The priority of the PCM is illustrated by an actual example in the supervised classification in this paper.