下载中心
优秀审稿专家
优秀论文
相关链接
摘要
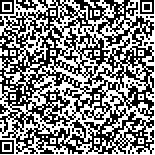
在传统的采用超平面作为判别边界的分割方法中,使用统计学的方法确定类判别界面,当区域间的统计值大于区域内的统计值时,就会出现类判决界面难于确定的问题。遗传算法的特点是进行全局优化搜索,利用这种算法优势可以解决传统算法难以解决的超平面空间定位问题,从而解决类判决界面的确定问题。通过EOS/MODIS图像数据的分割对该方法作了详细的介绍,其分类精度明显高于统计学分类方法。
For the traditional method of hyperplane segmentation, the location of hyperplane in data space was determined by statistical method. In the case of the statistical value of regions is smaller than thoes within the region, the statistical method was not effective. The character of genetic algorithm is global searching optimally. Taken this mathematical advantage, the location of Hyperplane could be located easily. In the paper, we use EOS/MODIS imagery data as an example to introduce this method in detail. We demonstrate that genetic algorithm can be used to produce segmentation result of remote sensing data. Using the parameters of the hyperplanes encoded in the chromosome, the region in which each training pattern point lies is determined by hyperplanes equation. Then the fitness is decided. After computing the fitness, the genetic operators of selection, crossover and mutation are applied to generate a new population of chromosomes. Then, after some process of this, the fitful hyperplane will be generated by this process. At last, the image was divided by the most fitful hyperplane equations. After the introduction of this method and its application, the comparative results with Maximum likelihood(ML)method in ERDAS IMAGINE 8.4 software package are given in section 4.As the paper shows, the genetic method is clearly better than ML method. It need to note that the realization is based on the Windows and Turbo c 2.0. We would have better results in VC++.based on Windows XP.