下载中心
优秀审稿专家
优秀论文
相关链接
摘要
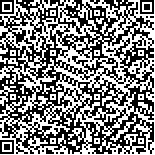
K-means聚类要求每个像素要和所有聚类中心求欧氏距离,当聚类数很多时,这是一个相当耗时的工作。改进的K—meam聚类算法根据历史聚类结果进行初始类分割,即节约初始聚类时间,又能使历史聚类过程中形成的类间稳定关系得以保持;类内像素只和相邻的聚类中心计算距离进行聚类,随着算法的迭代进行,大量类的状态基本固定,使得聚类速度不断加快。基于改进K-means聚类的无损压缩算法具有充分利用历史聚类成果和收敛速度快的特点,通过提高类内像素冗余度,最大限度消除谱间冗余和空间冗余。采用多次聚类压缩的结果预测最佳聚类数的方法,可实现最小熵无损压缩。通过和DPCM算法概率模型的熵值比较及实验数据的分析,验证了基于聚类无损压缩效率比不聚类无损压缩效果更优。
关键词:
超谱图像 无损压缩 熵 K-means 聚类Every pixel in the super space is required by K-means algorithm to calculate Euclidean distance for clustering.When there are many class centers,this is a rather time consuming work.In this paper,an improved K-means clustering algorithm is presented to save initial clustering time by making initial division based on previous clustering results,to remain the stable relationship between classes,and to accelerate clustering process with more and more classes becoming stable by judging the centers nearest to the pixel.A new clustering lossless compression algorithm designed here can determine the best class number and the highest compression ratio by fully utilizing previous clustering results and converging quickly eliminating the inter-spectral redundancy and intra-intra-spectral redundancy through enhancing the intra-class pixel redundancy.The convergence of this algorithm and existence of the best parameters are also inferred by making a deep analysis of the probability distribution model of the residue data.Furthermore,the comparison with DPCM lossless compression algorithm in the entropy value of the probability distribution model and the experimental results show that this clustering algorithm is better than non-clustering compression algorithm.Several times clustering approach can forecast the best class number with the least entropy lossless compression.