下载中心
优秀审稿专家
优秀论文
相关链接
摘要
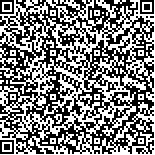
采用热红外多波段遥感数据反演陆面温度(LST),由于波段间信息高度相关,以及难以直接反演混合像元组分温度,使得LST的反演精度和应用价值都受到很大的限制。在建立非同温混合像元热辐射方向性模型基础上指出,热红外多角度遥感提供了反演组分温度的可能性,但是,由于该模型是采用蒙特卡洛方法模拟而建立起来的数值概念模型,采用一般反演方法很难同时提取所有参数信息。为了有效获取各参数信息,使用神经网络模型。由于待反演参数中,组分温度、土壤比辐射率和叶面积指数(LAI)都是多角度辐射亮度的非线性函数,然而,使用经典的误差后传(BP)算法容易陷入局部最优解区域;虽然遗传算法(GA)可以搜索到全局最优解,但在微机上实现算法速度太慢,因此,采用GA训练神经网络,得到网络权重,然后再以GA训练得到的权重作为BP算法的初始权重,继续训练神经网络,直到获得满意结果。这样既可以发挥BP算法快速寻优的特点,又能得到网络权重的最优组合。数值模拟的结果表明,基于非同温混合像元热辐射方向性模型,采用GA优化的神经网络模型反演多维参数效果比较理想。
关键词:
多角度热红外遥感 混合像元热辐射方向性模型 遗传算法 神经网络After carefully studying the results of retrieval of land surface temperature (LST) acquired by multi-channel thermal infrared remote sensing data, the authors point out that the accuracy and significance for applications are seriously damaged by high correlation coefficient among multi-channel information and its disablement of direct retrieval of component temperature. Based on the model of directional radiation of non-isothermal mixed pixel, we point out that the mufti-angle thermal infrared remote sensing can offer the possibility to directly retrieve component temperature. But it is difficult to synchronously retrieve all parameters using traditional inversion methods because the model is a numerical conception model based on Monte Carlo simulation. In order to effectively derive the parameters, we use neural network model. The parameters to be retrieved, such as component temperatures, soil emissivity and LAI,are all nonlinear function of mufti-angle radiation, and when the classical back-propagation (BP) algorithm was employed to retrieve these parameters, it was easily entrapped at local optimal regions. Therefore, we first employ genetic algorithm (GA) to train the neural network, and obtain the weights between the layers of neural network, which were used as the initial weights of the BP algorithm, then continue to train the network until the results are satisfied. Thus, the speediness of BP algorithm was developed and the optimal configuration of network weights are obtained. Based on the model of iadiant directionality of non-isothermal mixed pixel, simulated results show that retrieved mufti-dimensional parameters are superior when using GA to optimize the neural network weights.