摘要
热带与亚热带拥有大量丰富的自然资源,同时也正在经历着快速的城市化进程,其资源、生态、环境等都面临着前所未有的挑战。同时,热带与亚热带地区存在着大量的自然灾害(如台风、干旱、地震等),威胁着该地区人类经济社会的可持续发展。应用遥感技术对热带与亚热带区域进行全面的监测,对于热带与亚热带区域甚至全球的可持续发展具有重要的意义。然而,由于热带与亚热带特殊的地理条件(如多云多雨等),遥感监测需要克服特殊的技术挑战。本文通过Web of Science核心数据库的7594篇研究论文进行分析,综述了热带与亚热带遥感的研究现状,分别从热带与亚热带遥感的需求、现状、挑战和机遇,通过共被引文献分析和主题词频率分析,建立共被引文献网络和主题词网络,并通过非监督机器学习进行聚类,分别识别出22个共被引文献聚类和6个主题词聚类。通过对这些共被引文献类别和主题词类别的深入分析,本文总结了:(1)热带与亚热带遥感研究的主要监测对象,包括城市地表、热带雨林、红树林、珊瑚、热带草原、生物多样性和自然灾害;(2)热带与亚热带遥感主要采用的遥感技术,包括:遥感数据的选择和使用、遥感数据分析的方法、多云多雨的问题应对以及多源遥感技术。最后,从现代遥感技术的快速发展,本文从8个方面讨论热带与亚热带遥感面临的挑战和未来发展的机遇。
热带地区位于南北回归线之间,为太阳可以直射的区域,亚热带地区大致为南北纬40°与南北回归线(南北纬23°26′)之间的区域。然而,由于受到地形地貌、海陆位置等因素的影响,并非南北纬40°之间的所有区域都属于热带与亚热带气候,其具体的分布可以参考Köppen气候分类 (
由于热带与亚热带地区特殊的自然地理条件、人文地理特征、社会经济发展现状和可持续发展需求,应用现代遥感技术对热带亚热带地区的自然资源和人类活动进行辅助监测,对该区域乃至全球的可持续发展具有重要的意义,因为,过去近半个世纪以来,已有众多的遥感技术被应用于该区域的监测中。然后,由于热带与亚热带地区特殊的自然地理条件,遥感技术在该地区的有效应用也具有一定的区域性,需要解决一些具有明显区域特征的难题,如多云多雨、山脉众多、水系复杂等地理特征给传统的遥感技术带来了巨大的挑战。然而,尽管经历了近半个世纪的发展,已有的热带与亚热带地区遥感应用的案例,还比较分散,大部分已有的研究都是针对本地的某一个问题,采用某一种可获得的遥感数据进行数据分析和应用,这些研究方法常常难以直接应用到大范围的热带与亚热带区域,或者应用到其他亚热与亚热带区域。因此,需要对热带与亚热带遥感的研究现状做一次综合的梳理,弄清楚目前遥感技术在热带与亚热带地区的应用情况,包括遥感应用的领域,遥感监测的目标,遥感监测所使用的传感器和数据处理方法,以及目前所存在的问题。只有深入理解这些问题,梳理出热带与亚热带遥感应用的现状,总结出目前存在的问题,才能更好地、有针对性地分析这些问题,从而提出相应的解决方案,更好地支撑热带亚热带地区乃至全球的可持续发展。
本研究按照Köppen气候分类中热带与亚热带地区所覆盖的地理范围,列出热带与亚热带地区所包含的国家和地区。其中,如果某个国家整体属于这个地区,则列出国家名,如果该国家只有一部分属于这个地区,则列出这个部分的名字,可能是省份名字(如广东省)、城市名字(如香港、广州)或特殊地区名字(如亚马孙热带雨林、非洲大草原)等,因此,共列出了热带与亚热带地区223个地名。为了检索出具有代表性的热带与亚热带区域遥感应用的文章,本文选择Web of Science中的核心数据库(Core Collection)作为文献检索的数据库。其次,本研究将检索文献的范围限定在遥感领域,即Web of Science所划定的遥感领域(Remote Sensing)。然后,以上述223个地名作为关键词,在每篇文章的主题(包括题目、关键字和摘要)中检索包含这223个地区的所有文章。在这个检索过程中,有两点假设需要说明:(1)并非所有的热带与亚热带遥感应用的文章都会出现所列出的223个地方名字,有些比较小尺度的研究可能会出现小地名,如一个公园、保护区、或者小县城小城市的名字,对于这些研究,假定大部分在其研究区描述中仍然会加上国家名,这样文献就能被包含进来。而如果没有加上国家名,文献则会被本研究忽略,本研究假定这些被忽略的文章数量较少,不会影响文献调查的整体结果。(2)本研究只在遥感领域的期刊中检索,这样会遗漏一些在其他领域的期刊上发表的遥感应用的文章,由于目前跨学科研究比较普遍,这些文章可能有相当的数量。然而,本研究假定大部分这些文章,都有相似的文章发表在遥感领域的期刊中,且如果某一项研究包含一定成分的遥感技术信息,该研究更大可能会被发表在遥感类的期刊中。因而,本文假设,遥感领域的期刊能够有效反应热带与亚热带遥感应用的现状。此外,还将对所搜索出的文献的参考文献列表进行详细分析,而这些参考文献则包含大量的非遥感领域的相关研究,这在一定程度上也可以弥补文献检索时漏掉的部分跨学科研究文献。
最后,采用以上的关键字在Web of Science Core Collection数据库中共检索出11350篇文章,其中包括7496篇研究论文(Article),3912篇会议论文(Conference Proceedings),86篇综述(Review),83篇编辑评论(Editorial Materials),70篇未正式发表的文章(Early Access),25篇普通评论(Notes),21篇会议摘要(Meeting Abstracts),12篇勘误说明(Correction),9篇通讯文章(Letter),2篇新闻,1篇数据库综述(Database Review)。由于本文主要关注研究性文章,因此只包含了研究论文、未正式发表的文章和通讯文章,最后得到7594篇文章,其中包括75篇高被引文章(Highly Cited in Field),2篇热点文章(Hot Papers in Field),2251篇开放获取文章(Open Access)。这7594篇文章一共被引用194348次,h-index为162。
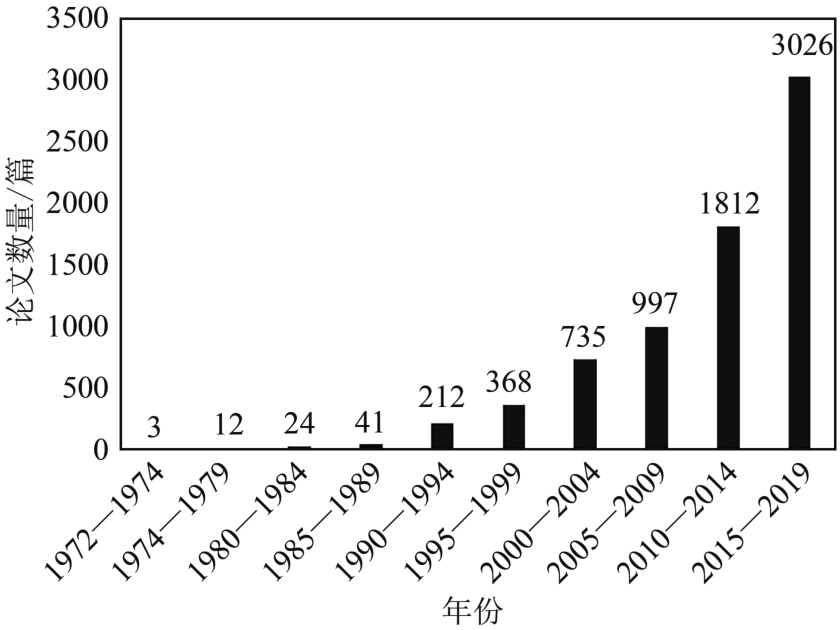
图1 热带与亚热带遥感文章1972年—2020年发表情况
Fig.1 Number of publications in tropical and subtropical remote sensing between 1972 and 2020
在文章引用方面,这7594篇文章被引用的次数也呈现稳步增长的趋势。如
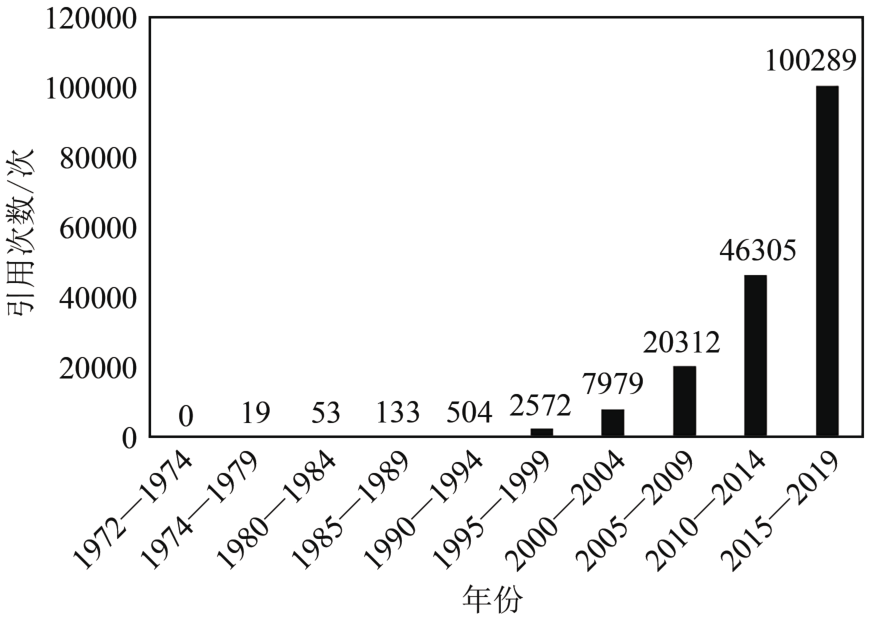
图2 热带与亚热带遥感文章1972年—2020年间被引用的情况
Fig.2 Yearly citations of the tropical and subtropical remote sensing publications between 1972 and 2020
为了全面分析这7594篇热带与亚热带遥感应用的文章,从而理解热带与亚热带遥感应用的现状,本研究采用两种文献分析方法。首先,本研究对所有文献进行共被引文献分析。通过对7594篇文献及其参考文献列表进行分析,如果某一篇参考文献列表中的文献(A)同时引用了7594篇文献中的两篇文献(B和C),则称B和C为共被引文献。如果B和C同时被一篇文献引用,则共被引强度为1,被N篇文献同时引用,则共被引强度为N。因此,可以对7594篇文献建立一个共被引关系图,即互为共被引关系的文献则建立一条边,边的权重为共被引强度。然后,对这个共被引关系图的结点进行聚类分析,可以划分成多个关系相对密切的聚类。对每一个聚类所包含的文章的主题进行分析,则可以识别这个聚类的研究主题。此外,还可以对每个聚类中所包含的文章进一步分析,例如文章发表的时间等。本研究对7594篇热带与亚热带遥感应用文章进行共被引分析,并对共被引关系图进行聚类,最终识别出22个聚类及其研究主题。

图3 热带与亚热带遥感共被引文献分析聚类(颜色代表类,共22类)
Fig.3 Co-citation analysis and clustering of tropical and subtropical remote sensing publications (each color for one cluster, 22 clusters in total)
对7594篇文章的题目和摘要中所包含的主题词进行分析。为了减少同一篇文章中重复多次出现某一个主题词而被重复统计,从而出现同一主题下有些文章被多次统计,而有些文章仅被统计一次的情况,本研究采用二元统计方法(binary counting),即如果一个词在一篇文章(题目+摘要)中出现过,则不管该词出现的次数,只记录为一次。最后,从7594篇文章中共识别出145488个主题词。为了减少某些统计分析的计算量,本研究只针对至少出现在10篇文章中的词进行统计分析,最后识别出符合条件的3907个主题词。在这3907个主题词中,有些主题词同时出现在同一篇文献中,这样就可以将这些主题词连接起来,从而建立一个关系网络,再对该关系网络进行聚类分析,则可以从该关系网络中分析出不同的聚类。最后,通过分析每一个聚类中所包含的主题词,可以评估每个聚类的大概主题和热点主题。

图4 热带与亚热带遥感文献题目和摘要词频分析与聚类(颜色代表类,共6类)
Fig.4 Term frequency analysis and clustering of tropical and subtropical remote sensing publications (each color for one cluster, six clusters in total)
城市地表与城市景观是热带与亚热带遥感监测的主要对象,在共被引文献分析聚类结果中,共有3个聚类与城市地表和景观的监测有关系,其中第1、7、8个聚类的主要监测对象包括城市地表,城市不透水面,农村—城市景观,城市地表温度,主要涉及多篇有代表性的研究论文 (
热带雨林是热带与亚热带遥感的重点和热点研究对象,由于热带雨林在全球碳循环中具有独特的作用,其在生态多样性保持等领域也有至关重要的作用,因此在过去几十年中不断受到各个国家和机构的关注和研究。在本研究中,共被引文献分析中的第2,15,28,54,17等几个聚类,以及文献主题词分析中的第4和第5个聚类,都同时涉及了热带雨林的研究。其主要的具体研究课题包括:热带次生林的监测 (
红树林的监测是热带与亚热带遥感应用的主要课题之一,在共被引文献聚类中的第6类和第10类,以及文献主题词分析聚类中的第5聚类都涉及到红树林的监测。该子领域的研究主要采用多源遥感技术,包括多波段多极化的SAR遥感、无人机遥感等,结合机器学习技术对红树林的树种进行识别分类,以及单株红树林识别,以支持红树林湿地保护 (
珊瑚是热带海域特殊的生态系统,由于其对水质和水温的变化非常敏感,被认为是全球变化的指示因子 (
热带草原气候又称为热带干湿季气候,热带草原是多种热带动物的重要栖息地,是重要的热带生态系统,因而也成为热带与亚热带遥感的重要应用领域之一。在本研究共被引文献聚类中第24和第40类,以及文献主题词分析中第1类都涉及到热带草原的监测,该子领域的研究也包含对热带与亚热带农作物的监测,主要的研究区分布在南非大草原 (
生物多样性是热带与亚热带生态系统的突出优势,不管是动物生态系统还是植物生态系统,其生态多样性都远远高于温带的动植物生态系统。然而,从本研究的文献分析结果来看,生物多样性的监测并非热带与亚热带遥感监测的直接对象之一。在文献共被引分析中,生物多样性监测没有被识别为单独的聚类。在主题词聚类分析中,第1聚类有部分文献涉及了热带与亚热带生物多样性的保护 (
随着近年来空气污染日益恶化,空气污染所引起的公共卫生问题日益受到关注,近20年来在热带与亚热带地区应用遥感技术监测和评估大气污染有关的问题也越来越多。该类研究主要与共被引文献分析聚类中的第13类紧密相关,包括采用光学遥感技术(如MODIS,AVHRR等)监测臭氧层的变化 (
自然灾害频繁发生是热带与亚热带地区的显著特征之一。由于特殊的地理位置和气候条件,热带与亚热带地区普遍都面临着多种自然灾害,包括热带气旋(台风),暴雨,洪水,干旱,山火等频发的自然灾害 (
从被引文献分析和文献主题词分析的结果来看,用于热带与亚热带遥感应用的卫星遥感数据仍然以中低分辨率的免费数据为主,特别是有NASA和USGS联合对外免费共享的Landsat系列卫星数据和MODIS卫星数据,这些数据可以支持区域尺度和全球尺度的热带与亚热带遥感监测,以及在一定程度上用于本地尺度的应用。此外,其他多光谱数据(如AVHRR),高光谱数据(如Hyperion),以及近年来快速兴起和普及的无人机遥感(UAV)数据,都被用于热带与亚热带监测中。包含这些不同数据的聚类包括共被引文献分析中的第3和第11个聚类,以及文献主题词分析中的第2个聚类 (
遥感数据的处理与分析是一个复杂的流程,包括遥感数据的预处理(大气校正和几何校正),地表生物物理参数的定量反演,地表土地覆盖与利用类型的识别、分类和变化监测等。这其中又涉及到有关电磁波辐射传输建模,地物生物物理过程模型建立,土地地表类型样本采集、特征提取与模式识别等。本研究通过对7594篇热带与亚热带遥感文献的共被引文献分析和主题词分析,发现不同的遥感数据分析方法都有学者在使用,这些方法被识别成主题词分析中的第1个聚类,其相关的主题词以图像分类为代表,包含了监督分类、非监督分类、面向对象分类、特征提取、分类模型,以及精度评估方法等多个研究主题词 (
多云多雨是热带与亚热带地区显著的气候特征,几乎存在于所有的热带与亚热带国家和地区中,这给遥感技术在热带与亚热带地区的有效应用带来了巨大的挑战,这一挑战集中表现为多云问题的应对与处理。尤其是光学遥感技术并不具备穿透云的能力,因而在有云遮挡的地方,光学遥感无法获取有效的影像数据,从而看不到或看不清想要监测的目标,大大影响了遥感监测的效果。在本研究的文献主题词分析结果中,第1和第2个聚类都表现出了云层处理这一关键词,这表明多云问题在热带与亚热带遥感中普遍存在,并成为了该地区遥感应用的一个关键问题 (
光学遥感受云层影响,大大降低了其在热带与亚热带地区数据获取能力,多源遥感技术自然而然地成为学者们考虑的一个解决思路。在已有的文献中,热带与亚热带遥感应用的主要多源遥感技术是采用合成孔径雷达(SAR)遥感,用于单独进行研究目标的监测,或者辅助传统的光学遥感,以提升遥感监测的效果。在本研究的共被引文献分析中,第12类显示了SAR以成为热带亚热带遥感应用的重要特征,并成为了一个独立的聚类。而在文献主题词的分析中,第4个聚类也直接与云层处理的关键字相关。SAR具有云层穿透能力,可全天候工作,这对于应对热带与亚热带区域多云多雨的气候特征是一项理想的遥感技术。热带与亚热带遥感应用所采用的SAR遥感技术包括:单极化SAR,多极化SAR和干涉SAR (
从热带与亚热带遥感研究的7594篇研究论文分析中可以看出,如何应对多云覆盖是热带与亚热带遥感在生态、环境、城市等各个领域的应用共同面对的技术挑战。因此在已有的文献中,各个领域的学者们都提出了多种应对热带与亚热带多云环境的技术手段和解决方案。另一方面,随着现代遥感技术的快速发展,不同的遥感平台、新型传感器和新的遥感数据处理与分析技术不断涌现,为热带与亚热带遥感应对云层覆盖的难题提供了大量的新机遇。本节将结合这些新的现代遥感技术,对热带与亚热带遥感面临的挑战进行讨论,分别包括光学遥感、雷达遥感、多源遥感融合、时间序列遥感技术、航空遥感和无人机遥感、新一代卫星计划与云计算平台等新技术。
光学遥感检测云覆盖处理技术是遥感影像质量提升的一个重要技术,包括处理光学遥感影像中存在的各种厚度和各种类型的云覆盖,以及云覆盖所形成的地面云阴影。该类技术一般包括云层检测与云层处理(修复或腌膜)两个步骤。一般而言,当云的厚度比较薄,部分地表信息仍然能被获取(例如通过近红外和热红外等受云影响较小的波段),云层可以通过某些技术去除,利用受影响较小的波段信息恢复其他波段的信息,从而复原光学影像;而当云层较厚,几乎所有波段都无法获取地面反射信息,则无法恢复受云覆盖影响的区域的信息。因此,通常需要对此类云层覆盖区域进行腌膜处理,或者采用其他数据源辅助复原。
由于受到多云多雨覆盖等因素的影响,热带与亚热带地区的光学遥感数据常常极其有限,且已有的具有长时间覆盖能力的光学遥感影像多为中低分辨率的卫星影响。这就使得在热带与亚热带遥感应用中需要处理包含大量混合像元的数据。混合像元分解技术使得从单一时相的影像中获取更多空间细节信息成为可能。这为热带与亚热带地区进行时间序列影像分析的研究(如亚马逊热带雨林和中非刚果盆地热带雨林的长时间演变)提供了重要的技术支撑。
合成孔径雷达遥感工作在波长范围约为几厘米到几十厘米(即微波)。相对于波长为几百纳米的可见光,微波具有穿透云层的能力,即便是厚云,微波也可以穿透。因此,即使在阴天情况下,SAR也可以获取地面清晰的影像,这使得SAR在恶劣天气下对地表进行全方位的监测具有独特的、其他遥感技术无法替代的优势。例如,在发生台风、暴雨、洪水、地震等自然灾害的时候,天气通常都很恶劣,而这些时候难以采用光学遥感对地表上的情况进行监测。因此,全面研究和推广SAR遥感技术在热带与亚热带区域的应用,对于热带与亚热带遥感应用,具有重要的现实意义。
由于单一的遥感数据源常常受到各种限制,如数据无法覆盖、受云层覆盖、受偶然的传感器故障等影响,从而无法对一个区域(尤其是较大尺度的区域)进行完整的监测与分析。因此,多源遥感融合是遥感应用一个重要的研究课题。已有的文献已经对多源遥感融合进行了全面而深入的研究,包括光学遥感与光学遥感的融合(如多光谱波段与全色波段的融合,不同多光谱遥感传感器之间的数据融合,多光谱与高光谱数据的融合),光学遥感与SAR遥感的融合,光学遥感与激光雷达遥感(LiDAR)的融合等。一般而言,光学、SAR、LiDAR之间属于多模态,即获取数据的机理不同,这类遥感数据之间的融合也称多模态遥感融合。如前所述,不同成像机理的遥感技术,常常能够最大程度地获取互补信息,因而多模态遥感融合成为了近年来的一个研究热点。多源多模态遥感融合技术通常包括像素级、特征级和决策级3种基本的融合层次。然而,尽管经过多年的发展,由于不同遥感技术之间的差异,以及地表信息的复杂情况,多源多模态遥感融合的技术仍然有大量的技术难点,在热带与亚热带遥感应用的课题中,仍然需要进行大量的科学研究。
时间序列遥感融合是近年来快速发展的一个领域,其主要源自应用遥感技术监测地表变化的现实需求。与上一节中的多源多模态遥感融合不同,时间序列遥感融合通常要求不同时间的数据来自同一传感器,或者同一类型的传感器。如果传感器不同,则需要对不同数据源进行预处理,例如处理成统一的空间分辨率。传统的时间序列遥感应用,包括对于地物历史长时序的演替分析,利用高时间分辨率低空间分辨率产品与低时间分辨率中高空间分辨率进行融合得到长时序高时间分辨率中高分辨率产品。主要应用于地表变化监测,例如地表土地覆盖土地利用变化(一般用时间序列光学遥感)和地表沉降监测(一般用干涉雷达遥感)。然而,时间序列遥感融合还可以用于数据修复和补充,包括:(1)采用同一时间内不同空间分辨率的光学遥感融合,可以补充高空间分辨率而低时间分辨率的数据,如Landsat数据与MODIS数据之间的时空融合。(2)针对某一类特殊区域,如被云层覆盖的区域,或者受阴影影响的区域,利用同一传感器在不同时间获取的同一位置的时间序列数据,进行云和阴影区域的信息修复。
随着近年来无人机的普及,航空遥感技术从专业领域走向了大众;又由于各行各业的专业应用(例如无人机应用于环境监测、生态监测、城市管理等),无人机使得航空遥感在各个领域应用的强大需求推动下,发展成为更加专业和完善的航空遥感技术。作为一个新型的传感器搭载平台,无人机可以搭载各种传感器,如多光谱传感器、高光谱传感器、LiDAR传感器等,从而满足不同领域的应用需求。在热带与亚热带遥感应用的过程中,无人机航空遥感意义更加非凡,例如即使无人机搭载了光学传感器,在多云的阴天情况下,由于其飞行位置在云层以下,仍然可以获取地面的信息。因此,无人机遥感已成为了许多地理、生态、环境行业野外作业过程中必备的信息获取手段。此外,由于无人机遥感独特的数据获取能力,将无人机遥感数据与传统的航空遥感数据及卫星遥感数据进行融合,从而促进卫星遥感数据的完善和提升大范围的地面监测能力,已成为现代遥感技术一个新的发展趋势,也为热带与亚热带遥感应用提供更大的技术支持和应用前景。
根据Zhou等(2010)的研究报告,对地观测卫星已经经历了5代:(1)第1代为早期卫星,主要是服务于冷战时期的区域制图需要,时间为1960年代初期到1972年。(2)第2代卫星为试验与初步应用,主要为全球资源管理提供制图,时间为1972年—1986年,这个时期的代表卫星是美国的Landsat 1。(3)第3代卫星是扩大应用领域,时间为1986年—1997年,代表性的卫星包括法国的SPOT-1和欧洲空间局的ERS-1。(4)第4代卫星为高分辨率卫星,时间为1997年—2010年。
本文3.1节从7594篇文章的共被引文献聚类和主题词聚类总结了热带与亚热带遥感应用主要的监测对象。然而,这些监测对象反映的是主要的研究热点和前沿科学问题,而并不能反映热带与亚热带遥感科学技术的系统性与综合性。在热带与亚热带遥感应用过程中,通过以上不同的监测对象,遥感技术与不同的学科进行了交叉融合,例如遥感与气候学(自然灾害、多云多雨等问题),遥感与生态学(红树林、珊瑚、热带草原、生物多样性等),遥感与水文学(自然灾害),遥感与环境科学(大气污染)等学科。遥感技术与这些学科交叉过程中更加促进了学科的发展,从而形成更全面的系统科学技术,对热带与亚热带地区的各种生态环境问题的理解和解决有重要的意义。
本文通过Web of Science核心数据库的7594篇研究论文进行分析,对共被引文献分析和主题词频率分析,分别识别出22个共被引文献聚类和6个主题词聚类。通过对这些共被引文献类别和主题词类别的深入分析,本文分别综述了热带与亚热带遥感研究的主要监测对象和主要遥感技术。针对现代遥感技术的快速发展趋势,本文讨论热带与亚热带遥感面临的挑战和未来发展的机遇,包括:(1)通过云检测与修复技术、以及混合像元分解技术为光学遥感更好地处理热带与亚热带遥感应用提供更好的技术支撑;(2)通过SAR雷达遥感的应用,以及对包括光学遥感与SAR遥感的多源多模态融合技术,为克服热带亚热带地区多云多雨问题带来新的机遇;(3)通过时间序列融合,对亚热带与亚热带地区进行长时间序列高时间分辨率和中高空间分辨率的遥感监测;(4)新一代卫星计划,包括中国、欧洲、美国、日本等的新一代卫星,以及近年来兴起的航空遥感与无人机遥感为热带与亚热带地区全面综合的监测提供更高新的技术和更可靠的观测数据,而云计算平台则促进了热带与亚热带地区全覆盖长时序的综合分析。(5)热带与亚热带遥感应用的深入促使多学科交叉形成更综合的系统科学技术。
参考文献(References)
Ajmar A, Boccardo P and Tonolo F G. 2011. Earthquake damage assessment based on remote sensing data. The Haiti case study. Italian Journal of Remote Sensing, 43(2): 123-128 [DOI: 10.5721/ItJRS20114329] [百度学术]
Alam K, Trautmann T, Blaschke T and Subhan F. 2014. Changes in aerosol optical properties due to dust storms in the Middle East and Southwest Asia. Remote Sensing of Environment, 143: 216-227 [DOI: 10.1016/j.rse.2013.12.021] [百度学术]
Asner G P, Carlson K M and Martin R E. 2005. Substrate age and precipitation effects on Hawaiian forest canopies from spaceborne imaging spectroscopy. Remote Sensing of Environment, 98(4): 457-467 [DOI: 10.1016/j.rse.2005.08.010] [百度学术]
Asner G P and Warner A S. 2003. Canopy shadow in IKONOS satellite observations of tropical forests and savannas. Remote Sensing of Environment, 87(4): 521-533 [DOI: 10.1016/j.rse.2003.08.006] [百度学术]
Avtar R, Kumar P, Oono A, Saraswat C, Dorji S and Hlaing Z. 2017. Potential application of remote sensing in monitoring ecosystem services of forests, mangroves and urban areas. Geocarto International, 32(8): 874-885 [DOI: 10.1080/10106049.2016.1206974] [百度学术]
Bagaram M B, Giuliarelli D, Chirici G, Giannetti F and Barbati A. 2018. UAV Remote Sensing for Biodiversity Monitoring: Are Forest Canopy Gaps Good Covariates?. Remote Sensing, 10: 1397 [DOI: 10.20944/preprints201807.0209.v1] [百度学术]
Beloconi A, Kamarianakis Y and Chrysoulakis N. 2016. Estimating urban PM10 and PM2.5 concentrations, based on synergistic MERIS/AATSR aerosol observations, land cover and morphology data. Remote Sensing of Environment, 172: 148-164 [DOI: 10.1016/j.rse.2015.10.017] [百度学术]
Blaschke T, Hay G J, Weng Q H and Resch B. 2011. Collective sensing: integrating geospatial technologies to understand urban systems-an overview. Remote Sensing, 3(8): 1743-1776 [DOI: 10.3390/rs3081743] [百度学术]
Bouvet A, Le Toan T and Lam-Dao N. 2009. Monitoring of the rice cropping system in the Mekong delta using ENVISAT/ASAR dual polarization data. IEEE Transactions on Geoscience and Remote Sensing, 47(2): 517-526 [DOI: 10.1109/TGRS.2008.200 7963] [百度学术]
Buitre M J C, Zhang H S and Lin H. 2019. The mangrove forests change and impacts from tropical cyclones in the Philippines using time series satellite imagery. Remote Sensing, 11(6): 688 [DOI: 10.3390/rs11060688] [百度学术]
Cárdenas N Y, Joyce K E and Maier S W. 2017. Monitoring mangrove forests: are we taking full advantage of technology?. International Journal of Applied Earth Observation and Geoinformation, 63: 1-14 [DOI: 10.1016/j.jag.2017.07.004] [百度学术]
Chai D F, Newsam S, Zhang H K K, Qiu Y F and Huang J F. 2019. Cloud and cloud shadow detection in Landsat imagery based on deep convolutional neural networks. Remote Sensing of Environment, 225: 307-316 [DOI: 10.1016/j.rse.2019.03.007] [百度学术]
Chen B Q, Xiao X M, Li X P, Pan L H, Doughty R, Ma J, Dong J W, Qin Y W, Zhao B, Wu Z X, Sun R, Lan G Y, Xie G S, Clinton N and Giri C. 2017. A mangrove forest map of China in 2015: Analysis of time series Landsat 7/8 and Sentinel-1A imagery in Google Earth Engine cloud computing platform. ISPRS Journal of Photogrammetry and Remote Sensing, 131: 104-120 [DOI: 10.1016/j.isprsjprs.2017.07.011] [百度学术]
Chen H N, Chandrasekar V, Cifelli R and Xie P P. 2020. A machine learning system for precipitation estimation using satellite and ground radar network observations. IEEE Transactions on Geoscience and Remote Sensing, 58(2): 982-994 [DOI: 10.1109/TGRS.2019.2942280] [百度学术]
陈述彭, 周成虎, 陆锋. 2005. 季风热带与亚热带地区的遥感应用实验. 地球信息科学, 7(4): 2-4 [百度学术]
Chen S P, Zhou C H and Lu F. 2005. Several remote sensing experiments in monsoon tropical and subtropical regions. Geo-Information Science, 7(4): 2-4 [百度学术]
Choi W, Lee H, Kim J, Ryu J Y, Park S S, Park J and Kang H. 2019. Effects of spatiotemporal O4 column densities and temperature-dependent O4 absorption cross-section on an aerosol effective height retrieval algorithm using the O4 air mass factor from the ozone monitoring instrument. Remote Sensing of Environment, 229: 223-233 [DOI: 10.1016/j.rse.2019.05.001] [百度学术]
Choi Y and Souri A H. 2015. Chemical condition and surface ozone in large cities of Texas during the last decade: observational evidence from OMI, CAMS, and model analysis. Remote Sensing of Environment, 168: 90-101 [DOI: 10.1016/j.rse.2015.06.026] [百度学术]
Collin A, Nadaoka K and Bernardo L. 2015. Mapping the socio-economic and ecological resilience of Japanese coral reefscapes across a decade. ISPRS International Journal of Geo-Information, 4(2): 900-927 [DOI: 10.3390/ijgi4020900] [百度学术]
Dimitrov P, Dong Q H, Eerens H, Gikov A, Filchev L, Roumenina E and Jelev G. 2019. Sub-pixel crop type classification using PROBA-V 100 m NDVI time series and reference data from sentinel-2 classifications. Remote Sensing, 11: 1370 [DOI: 10.3390/rs11111370] [百度学术]
Dong J W, Xiao X M, Sheldon S, Biradar C and Xie G S. 2012. Mapping tropical forests and rubber plantations in complex landscapes by integrating PALSAR and MODIS imagery. ISPRS Journal of Photogrammetry and Remote Sensing, 74: 20-33 [DOI: 10.1016/j.isprsjprs.2012.07.004] [百度学术]
Dong L G and Shan J. 2013. A comprehensive review of earthquake-induced building damage detection with remote sensing techniques. ISPRS Journal of Photogrammetry and Remote Sensing, 84: 85-99 [DOI: 10.1016/j.isprsjprs.2013.06.011] [百度学术]
Doren R F, Rutchey K and Welch R. 1999. The Everglades: a perspective on the requirements and applications for vegetation map and database products. Photogrammetric Engineering and Remote Sensing, 65(2): 155-161 [百度学术]
Dupuis C, Lejeune P, Michez A and Fayolle A. 2020. How can remote sensing help monitor tropical moist forest degradation?-A systematic review. Remote Sensing, 12(7): 1087 [DOI: 10.3390/rs12071087] [百度学术]
Fonseka H P U, Zhang H S, Sun Y, Su H, Lin H and Lin Y Y. 2019. Urbanization and its impacts on land surface temperature in Colombo metropolitan area, Sri Lanka, from 1988 to 2016. Remote Sensing, 11(8):957 [DOI: 10.3390/rs11080957] [百度学术]
Friess D A and Webb E L. 2014. Variability in mangrove change estimates and implications for the assessment of ecosystem service provision. Global Ecology and Biogeography, 23: 715-725 [DOI: 10.1111/geb.12140] [百度学术]
Gagliardini D A, Clemente-Colón P, Bava J, Milovich J A and Frulla L A. 2001. Complementary use of SAR and thermal IR observations in the Brazil-Malvinas confluence region. Canadian Journal of Remote Sensing, 27(6): 643-650 [DOI: 10.1080/07038992.2001.10854906] [百度学术]
Gastellu-Etchegorry J P. 1988. Cloud cover distribution in Indonesia. International Journal of Remote Sensing, 9(7): 1267-1276 [DOI: 10.1080/01431168808954934] [百度学术]
Geng G N, Zhang Q, Martin R V, van Donkelaar A, Huo H, Che H Z, Lin J T and He K B. 2015. Estimating long-term PM2.5 concentrations in China using satellite-based aerosol optical depth and a chemical transport model. Remote Sensing of Environment, 166: 262-270 [百度学术]
Giri C, Ochieng E, Tieszen L L, Zhu Z, Singh A, Loveland T, Masek J and Duke N. 2011. Status and distribution of mangrove forests of the world using earth observation satellite data. Global Ecology and Biogeography, 20: 154-159 [DOI: 10.1111/j.1466-8238.2010.00584.x] [百度学术]
Giuliani G and Peduzzi P. 2011. The PREVIEW Global Risk Data Platform: a geoportal to serve and share global data on risk to natural hazards. Natural Hazards and Earth System Sciences, 11: 53-66 [DOI: 10.5194/nhess-11-53-2011] [百度学术]
Goodwin N R, Collett L J, Denham R J, Flood N and Tindall D. 2013. Cloud and cloud shadow screening across Queensland, Australia: an automated method for Landsat TM/ETM + time series. Remote Sensing of Environment, 134: 50-65 [DOI: 10.1016/j.rse.2013.02.019] [百度学术]
Green E P, Clark C D, Mumby P J, Edwards A J and Ellis A C. 1998. Remote sensing techniques for mangrove mapping. International Journal of Remote Sensing, 19(5): 935-956 [DOI: 10.1080/014311698215801] [百度学术]
Grishin G A and Savoskin, V M. 1995. Simulation of the influence of clouds on the outgoing long-wave-radiation from Noaa-11 satellite data. Earth Observation and Remote Sensing, 12: 737-743 [百度学术]
Guo H D, Goodchild M F and Annoni A. 2020. Manual of Digital Earth. Singapore: Springer [DOI: 10.1007/978-981-32-9915-3] [百度学术]
Guo M, Li J, Sheng C L, Xu J W and Wu L. 2017. A review of wetland remote sensing. Sensors, 17(4): 777 [DOI: 10.3390/s17040777] [百度学术]
Hansen M C and Loveland T R. 2012. A review of large area monitoring of land cover change using Landsat data. Remote Sensing of Environment, 122: 66-74 [DOI: 10.1016/j.rse.2011.08.024] [百度学术]
Hedley J D, Roelfsema C, Brando V, Giardino C, Kutser T, Phinn S, Mumby P J, Barrilero O, Laporte J and Koetz B. 2018. Coral reef applications of Sentinel-2: coverage, characteristics, bathymetry and benthic mapping with comparison to Landsat 8. Remote Sensing of Environment, 216: 598-614 [DOI: 10.1016/j.rse.2018.07.014] [百度学术]
Hedley J D, Roelfsema C M, Chollett I, Harborne A R, Heron S F, Weeks S, Skirving W J, Strong A E, Eakin C M, Christensen T R L, Ticzon V, Bejarano S and Mumby P J. 2016. Remote sensing of coral reefs for monitoring and management: a review. Remote Sensing, 8(2): 118 [DOI: 10.3390/rs8020118] [百度学术]
Hedley J D, Roelfsema C M, Phinn S R and Mumby P J. 2012. Environmental and sensor limitations in optical remote sensing of coral reefs: implications for monitoring and sensor design. Remote Sensing, 4(1): 271-302 [DOI: 10.3390/rs4010271] [百度学术]
Hethcoat M G, Edwards D P, Carreiras J M B, Bryant R G, Franca F M and Quegan S. 2019. A machine learning approach to map tropical selective logging. Remote Sensing of Environment, 221: 569-582 [DOI: 10.1016/j.rse.2018.11.044] [百度学术]
Higginbottom T P, Symeonakis E, Meyer H and van der Linden S. 2018. Mapping fractional woody cover in semi-arid savannahs using multi-seasonal composites from Landsat data. ISPRS Journal of Photogrammetry and Remote Sensing, 139: 88-102 [DOI: 10.1016/j.isprsjprs.2018.02.010] [百度学术]
Honda K and Nagai M. 2002. Real-time volcano activity mapping using ground-based digital imagery. ISPRS Journal of Photogrammetry and Remote Sensing, 57(1/2): 159-168 [DOI: 10.1016/S0924-2716(02)00112-0] [百度学术]
Hu L J, Li W Y and Xu B. 2018. The role of remote sensing on studying mangrove forest extent change. International Journal of Remote Sensing, 39(19): 6440-6462 [DOI: 10.1080/01431161.2018.1455239] [百度学术]
Jensen J R, Qiu F and Ji M H. 1999. Predictive modelling of coniferous forest age using statistical and artificial neural network approaches applied to remote sensor data. International Journal of Remote Sensing, 20(14): 2805-2822 [DOI: 10.1080/0143116 99211804] [百度学术]
Jia M M, Wang Z M, Wang C, Mao D H and Zhang Y Z. 2019. A new vegetation index to detect periodically submerged mangrove forest using single-tide sentinel-2 imagery. Remote Sensing, 11(17): 2043 [DOI: 10.3390/rs11172043] [百度学术]
Jiang L M, Liao M S, Lin H and Yang L M. 2009. Synergistic use of optical and InSAR data for urban impervious surface mapping: a case study in Hong Kong. International Journal of Remote Sensing, 30(11): 2781-2796 [DOI: 10.1080/01431160802555838] [百度学术]
Jiang X, Liu Y, Yu B and Jiang M. 2007. Comparison of MISR aerosol optical thickness with AERONET measurements in Beijing metropolitan area. Remote Sensing of Environment, 107(1/2): 45-53 [DOI: 10.1016/j.rse.2006.06.022] [百度学术]
Johannessen J A. 1999. Marine coastal ocean monitoring: SAR in synergy with other remote sensing observations//Proceedings of the Euro-Asian Space Week on Cooperation in Space. Singapore, 430: 311-319 [百度学术]
Kaufman Y J and Gao B C. 1992. Remote sensing of water vapor in the near IR from EOS/MODIS. IEEE Transactions on Geoscience and Remote Sensing, 30(5): 871-884 [DOI: 10.1109/36.175321] [百度学术]
Kaufman Y J, Tanré D and Boucher O. 2002. A satellite view of aerosols in the climate system. Nature, 419(6903): 215-223 [DOI: 10.1038/nature01091] [百度学术]
Kazansky A V. 1997. Dual-path method and revised radiative model for retrieving cloud parameters from pairs of AVHRR images. Remote Sensing of Environment, 59(3): 417-427 [DOI: 10.1016/0034-4257(95)00101-8] [百度学术]
Kim W, Kim J, Jung Y, Boesch H, Lee H, Lee S, Goo T Y, Jeong U, Kim M, Cho C H and Ou M L. 2016. Retrieving XCO2 from GOSAT FTS over East Asia using simultaneous aerosol information from CAI. Remote Sensing, 8(12): 994 [DOI: 10.3390/rs8120994] [百度学术]
Kimes D S, Nelson R F, Salas W A and Skole D L. 1999. Mapping secondary tropical forest and forest age from SPOT HRV data. International Journal of Remote Sensing, 20(18): 3625-3640 [DOI: 10.1080/014311699211246] [百度学术]
Knudby A, LeDrew E and Newman C. 2007. Progress in the use of remote sensing for coral reef biodiversity studies. Progress in Physical Geography, 31(4): 421-434 [DOI: 10.1177/030913330708 1292] [百度学术]
Kottek M, Grieser J, Beck C, Rudolf B and Rubel F. 2006. World map of the Köppen-Geiger climate classification updated. Meteorologische Zeitschrift, 15(3): 259-263 [DOI: 10.1127/0941-2948/2006/0130] [百度学术]
Kovacs J M, Lu X X, Flores-Verdugo F, Zhang C, de Santiago F F and Jiao X. 2013. Applications of ALOS PALSAR for monitoring biophysical parameters of a degraded black mangrove (Avicennia germinans) forest. ISPRS Journal of Photogrammetry and Remote Sensing, 82: 102-111 [DOI: 10.1016/j.isprsjprs.2013.05.004] [百度学术]
Kuenzer C, Bluemel A, Gebhardt S, Quoc T V and Dech S. 2011. Remote sensing of mangrove ecosystems: a review. Remote Sensing, 3(5): 878-928 [DOI: 10.3390/rs3050878] [百度学术]
Lee J, Kim J, Song C H, Ryu J H, Ahn Y H and Song C K. 2010. Algorithm for retrieval of aerosol optical properties over the ocean from the Geostationary Ocean Color Imager. Remote Sensing of Environment, 114(5): 1077-1088 [DOI: 10.1016/j.rse.2009.12.021] [百度学术]
Li X, Yeh A G O, Wang S, Liu K, Liu X, Qian J and Chen X. 2007. Regression and analytical models for estimating mangrove wetland biomass in South China using Radarsat images. International Journal of Remote Sensing, 28(24): 5567-5582 [DOI: 10.1080/01431160701227638] [百度学术]
Li Z L and Becker F. 1993. Feasibility of land surface temperature and emissivity determination from AVHRR data. Remote Sensing of Environment, 43(1): 67-85 [DOI: 10.1016/0034-4257(93)90065-6] [百度学术]
Liew S C, Kam S P, Tuong T P, Chen P, Minh V Q and Lim H. 1998. Application of multitemporal ERS-2 synthetic aperture radar in delineating rice cropping systems in the Mekong River Delta, Vietnam. IEEE Transactions on Geoscience and Remote Sensing, 36(5): 1412-1420 [DOI: 10.1109/36.718845] [百度学术]
Lin C A, Chen Y C, Liu C Y, Chen W T, Seinfeld J H and Chou C C K. 2019. Satellite-derived correlation of SO2, NO2, and aerosol optical depth with meteorological conditions over East Asia from 2005 to 2015. Remote Sensing, 11(15): 1738 [DOI: 10.3390/rs11151738] [百度学术]
Lin Y Y, Zhang H S, Lin H, Gamba P E and Liu X P. 2020. Incorporating synthetic aperture radar and optical images to investigate the annual dynamics of anthropogenic impervious surface at large scale. Remote Sensing of Environment, 242: 111757 [DOI: 10.1016/j.rse.2020.111757] [百度学术]
Liu M F, Zhang H S, Lin G H, Lin H and Tang D L. 2018. Zonation and directional dynamics of mangrove forests derived from time-series satellite imagery in Mai Po, Hong Kong. Sustainability, 10(6): 1913 [DOI: 10.3390/su10061913] [百度学术]
Liu Y, Franklin M, Kahn R and Koutrakis P. 2007. Using aerosol optical thickness to predict ground-level PM2.5 concentrations in the St. Louis area: a comparison between MISR and MODIS. Remote Sensing of Environment, 107(1/2): 33-44 [DOI: 10.1016/j.rse.2006.05.022] [百度学术]
Luo L and Mountrakis G. 2010. Integrating intermediate inputs from partially classified images within a hybrid classification framework: an impervious surface estimation example. Remote Sensing of Environment, 114(6): 1220-1229 [DOI: 10.1016/j.rse.2010.01.008] [百度学术]
Luo L and Mountrakis G. 2011. Converting local spectral and spatial information from a priori classifiers into contextual knowledge for impervious surface classification. ISPRS Journal of Photogrammetry and Remote Sensing, 66(5): 579-587 [DOI: 10.1016/j.isprsjprs.2011.03.002] [百度学术]
Mahrooghy M, Aanstoos J V, Nobrega R A A, Hasan K, Prasad S and Younan N H. 2015. A machine learning framework for detecting landslides on earthen levees using spaceborne SAR imagery. IEEE Journal of Selected Topics in Applied Earth Observations and Remote Sensing, 8(8): 3791-3801 [DOI: 10.1109/JSTARS.2015.2427337] [百度学术]
Malingreau J P and Belward A S. 1994. Recent activities in the European Community for the creation and analysis of global AVHRR data sets. International Journal of Remote Sensing, 15(17): 3397-3416 [DOI: 10.1080/01431169408954337] [百度学术]
Malingreau J P and Tucker C J. 1990. Ranching in the Amazon Basin - Large-scale changes observed by AVHRR. International Journal of Remote Sensing, 11(2): 187-189 [DOI: 10.1080/014311690 08955012] [百度学术]
Manavalan R. 2018. Review of synthetic aperture radar frequency, polarization, and incidence angle data for mapping the inundated regions. Journal of Applied Remote Sensing, 12(2): 021501 [DOI: 10.1117/1.JRS.12.021501] [百度学术]
Martins V S, Novo E M L M, Lyapustin A, Aragão L E O C, Freitas S R and Barbosa C C F. 2018. Seasonal and interannual assessment of cloud cover and atmospheric constituents across the Amazon (2000-2015): insights for remote sensing and climate analysis. ISPRS Journal of Photogrammetry and Remote Sensing, 145: 309-327 [DOI: 10.1016/j.isprsjprs.2018.05.013] [百度学术]
Maselli F, Papale D, Chiesi M, Matteucci G, Angeli L, Raschi A and Seufert G. 2014. Operational monitoring of daily evapotranspiration by the combination of MODIS NDVI and ground meteorological data: application and evaluation in Central Italy. Remote Sensing of Environment, 152: 279-290 [DOI: 10.1016/j.rse.2014.06.021] [百度学术]
Mason R A B, Skirving W J and Dove S G. 2020. Integrating physiology with remote sensing to advance the prediction of coral bleaching events. Remote Sensing of Environment, 246: 111794 [DOI: 10.1016/j.rse.2020.111794] [百度学术]
Mathieu R, Naidoo L, Cho M A, Leblon B, Main R, Wessels K, Asner G P, Buckley J, Van Aardt J, Erasmus B F N and Smit I P J. 2013. Toward structural assessment of semi-arid African savannahs and woodlands: the potential of multitemporal polarimetric RADARSAT-2 fine beam images. Remote Sensing of Environment, 138: 215-231 [DOI: 10.1016/j.rse.2013.07.011] [百度学术]
Miller M E, Lefsky M and Pang Y. 2011. Optimization of Geoscience Laser Altimeter System waveform metrics to support vegetation measurements. Remote Sensing of Environment, 115(2): 298-305 [DOI: 10.1016/j.rse.2010.09.002] [百度学术]
Minnis P, Sun-Mack S, Young D F, Heck P W, Garber D P, Chen Y, Spangenberg D A, Arduini R F, Trepte Q Z, Smith W L, Ayers J K, Gibson S C, Miller W F, Hong G, Chakrapani V, Takano Y, Liou K N, Xie Y and Yang P. 2011. CERES edition-2 cloud property retrievals using TRMM VIRS and terra and aqua MODIS data-Part I: algorithms. IEEE Transactions on Geoscience and Remote Sensing, 49(11): 4374-4400 [DOI: 10.1109/TGRS.2011.2144601] [百度学术]
Mohamad I N, Hayashi T, Uyeda H, Terao T and Kikuchi K. 2004. Diurnal variations of cloud activity in Bangladesh and north of the Bay of Bengal in 2000. Remote Sensing of Environment, 90(3): 378-388 [DOI: 10.1016/j.rse.2004.01.011] [百度学术]
Myint S W, Giri C P, Le W, Zhu Z L and Gillette S C. 2008. Identifying mangrove species and their surrounding land use and land cover classes using an object-oriented approach with a lacunarity spatial measure. Giscience and Remote Sensing, 45(2): 188-208 [DOI: 10.2747/1548-1603.45.2.188] [百度学术]
Okoro E and Okeke F. 2017. Effects of zonal wind on stratospheric ozone variations over Nigeria. International Journal of Remote Sensing, 38(6): 1665-1681 [DOI: 10.1080/01431161.2017.1286053] [百度学术]
Otukei J R and Blaschke T. 2010. Land cover change assessment using decision trees, support vector machines and maximum likelihood classification algorithms. International Journal of Applied Earth Observation and Geoinformation, 12(S1): S27-S31 [DOI: 10.1016/j.jag.2009.11.002] [百度学术]
Palandro D A, Andréfouët S, Hu C M, Hallock P, Müller-Karger F E, Dustan P, Callahan M K, Kranenburg C and Beaver C R. 2008. Quantification of two decades of shallow-water coral reef habitat decline in the Florida Keys National Marine Sanctuary using Landsat data (1984-2002). Remote Sensing of Environment, 112(8): 3388-3399 [DOI: 10.1016/j.rse.2008.02.015] [百度学术]
Pettorelli N, Vik J O, Mysterud A, Gaillard J M, Tucker C J and Stenseth N C. 2005. Using the satellite-derived NDVI to assess ecological responses to environmental change. Trends in Ecology and Evolution, 20(9): 503-510 [DOI: 10.1016/j.tree.2005.05.011] [百度学术]
Pham T D, Yokoya N, Bui D T, Yoshino K and Friess D A. 2019. Remote sensing approaches for monitoring mangrove species, structure, and biomass: opportunities and challenges. Remote Sensing, 11(3): 230 [DOI: 10.3390/rs11030230] [百度学术]
Pham T D, Yokoya N, Xia J S, Ha N T, Le N N, Nguyen T T T, Dao T H, Vu T T P, Pham T D and Takeuchi W. 2020. Comparison of machine learning methods for estimating mangrove above-ground biomass using multiple source remote sensing data in the Red River Delta Biosphere Reserve, Vietnam. Remote Sensing, 12(8): 1334 (OI: 10.3390/rs12081334) [百度学术]
Popov M A, Kussul N N, Stankevich S A, Kozlova A A, Shelestov A Y, Kravchenko O M, Korbakov M B and Skakun S V. 2008. Web service for biodiversity estimation using remote sensing data. International Journal of Digital Earth, 1(4): 367-376 [DOI: 10.1080/17538940802483745] [百度学术]
Prasad A K and Singh R P. 2007. Comparison of MISR-MODIS aerosol optical depth over the Indo-Gangetic basin during the winter and summer seasons (2000-2005). Remote Sensing of Environment, 107(1/2): 109-119 [DOI: 10.1016/j.rse.2006.09.026] [百度学术]
Racault M F, Raitsos D E, Berumen M L, Brewin R J W, Platt T, Sathyendranath S and Hoteit I. 2015. Phytoplankton phenology indices in coral reef ecosystems: application to ocean-color observations in the Red Sea. Remote Sensing of Environment, 160: 222-234 [DOI: 10.1016/j.rse.2015.01.019] [百度学术]
Ramsey E W III and Jensen J R. 1996. Remote sensing of mangrove wetlands: relating canopy spectra to site-specific data. Photogrammetric Engineering and Remote Sensing, 62(8): 939-948 [百度学术]
Renó V, Novo E and Escada M. 2016. Forest fragmentation in the Lower Amazon Floodplain: implications for biodiversity and ecosystem service provision to riverine populations. Remote Sensing, 8(11): 886 [DOI: 10.3390/rs8110886] [百度学术]
Reygadas Y, Jensen J L R and Moisen G G. 2019. Forest degradation assessment based on trend analysis of MODIS-leaf area index: a case study in Mexico. Remote Sensing, 11(21): 2503 [DOI: 10.3390/rs11212503] [百度学术]
Rignot E, Salas W A and Skole D L. 1997. Mapping deforestation and secondary growth in Rondonia, Brazil, using imaging radar and thematic mapper data. Remote Sensing of Environment, 59(2): 167-179 [DOI: 10.1016/S0034-4257(96)00150-2] [百度学术]
Rudorff N, Rudorff C M, Kampel M and Ortiz G. 2018. Remote sensing monitoring of the impact of a major mining wastewater disaster on the turbidity of the Doce River plume off the eastern Brazilian coast. ISPRS Journal of Photogrammetry and Remote Sensing, 145: 349-361 [DOI: 10.1016/j.isprsjprs.2018.02.013] [百度学术]
Russell A, Milford J, Bergin M S, McBride S, McNair L, Yang Y, Stockwell W R and Croes B. 1995. Urban ozone control and atmospheric reactivity of organic gases. Science, 269(5223): 491-495 [DOI: 10.1126/science.269.5223.491] [百度学术]
Rutchey K and Vilchek L. 1999. Air photointerpretation and satellite imagery analysis techniques for mapping cattail coverage in a northern Everglades impoundment. Photogrammetric Engineering and Remote Sensing, 65(2): 185-191 [百度学术]
Santos M J, Disney M and Chave J. 2018. Detecting human presence and influence on neotropical forests with remote sensing. Remote Sensing, 10(10): 1593 [DOI: 10.3390/rs10101593] [百度学术]
Segal-Rozenhaimer M, Li A, Das K and Chirayath V. 2020. Cloud detection algorithm for multi-modal satellite imagery using convolutional neural-networks (CNN). Remote Sensing of Environment, 237: 111446 [DOI: 10.1016/j.rse.2019.111446] [百度学术]
Sinyuk A, Dubovik O, Holben B, Eck T F, Breon F M, Martonchik J, Kahn R, Diner D J, Vermote E F, Roger J C, Lapyonok T and Slutsker I. 2007. Simultaneous retrieval of aerosol and surface properties from a combination of AERONET and satellite data. Remote Sensing of Environment, 107(1/2): 90-108 [DOI: 10.1016/j.rse.2006.07.022] [百度学术]
Smith L B and Wright J W. 1972. Sporadic-E and wind-profile interrelation over Hawaii. Radio Science, 7(3): 363-366 [DOI: 10.1029/RS007i003p00363] [百度学术]
Sobrino J A, Coll C and Caselles V. 1991. Atmospheric correction for land surface temperature using NOAA-11 AVHRR channels 4 and 5. Remote Sensing of Environment, 38(1): 19-34 [DOI: 10.1016/0034-4257(91)90069-I] [百度学术]
Stampoulis D, Haddad Z S and Anagnostou E N. 2014. Assessing the drivers of biodiversity in Madagascar by quantifying its hydrologic properties at the watershed scale. Remote Sensing of Environment, 148: 1-15 [DOI: 10.1016/j.rse.2014.03.005] [百度学术]
Thenkabail P S, Enclona E A, Ashton M S, Legg C and De Dieu M J. 2004. Hyperion, IKONOS, ALI, and ETM + sensors in the study of African rainforests. Remote Sensing of Environment, 90(1): 23-43 [DOI: 10.1016/j.rse.2003.11.018] [百度学术]
Trigg S N, Roy D P and Flasse S P. 2005. An in situ study of the effects of surface anisotropy on the remote sensing of burned savannah. International Journal of Remote Sensing, 26(21): 4869-4876 [DOI: 10.1080/01431160500141923] [百度学术]
United Nations Economic and Social Commission for Asia and the Pacific (UNESCAP). 2015. Disasters without borders: Regional resilience for sustainable development. Asia-Pacific Disaster Report 2015 [百度学术]
Wan Z M. 2014. New refinements and validation of the collection-6 MODIS land-surface temperature/emissivity product. Remote Sensing of Environment, 140: 36-45 [DOI: 10.1016/j.rse.2013.08.027] [百度学术]
Wan Z M, Zhang Y L, Zhang Q C and Li Z L. 2002. Validation of the land-surface temperature products retrieved from Terra Moderate Resolution Imaging Spectroradiometer data. Remote Sensing of Environment, 83(1/2): 163-180 [DOI: 10.1016/S0034-4257(02)00093-7] [百度学术]
Wang D, Lin H, Chen J S, Zhang Y Z and Zeng Q W. 2010. Application of multi-temporal ENVISAT ASAR data to agricultural area mapping in the Pearl River Delta. International Journal of Remote Sensing, 31(6): 1555-1572 [DOI: 10.1080/01431160903475258] [百度学术]
Wang L, Jia M M, Yin D M and Tian J Y. 2019. A review of remote sensing for mangrove forests: 1956-2018. Remote Sensing of Environment, 231: 111223 [DOI: 10.1016/j.rse.2019.111223] [百度学术]
Whittle M, Quegan S, Uryu Y, Stüewe M and Yulianto K. 2012. Detection of tropical deforestation using ALOS-PALSAR: a Sumatran case study. Remote Sensing of Environment, 124: 83-98 [DOI: 10.1016/j.rse.2012.04.027] [百度学术]
Wiens J, Sutter R, Anderson M, Blanchard J, Barnett A, Aguilar-Amuchastegui N, Avery C and Laine S. 2009. Selecting and conserving lands for biodiversity: the role of remote sensing. Remote Sensing of Environment, 113(7): 1370-1381 [DOI: 10.1016/j.rse.2008.06.020] [百度学术]
Xu R, Zhang H S and Lin H. 2017a. Urban impervious surfaces estimation from optical and SAR imagery: a comprehensive comparison. IEEE Journal of Selected Topics in Applied Earth Observations and Remote Sensing, 10(9): 4010-4021 [DOI: 10.1109/JSTARS.2017.2706747] [百度学术]
Xu R, Zhang H S, Wang T and Lin H. 2017b. Using pan-sharpened high resolution satellite data to improve impervious surfaces estimation. International Journal of Applied Earth Observation and Geoinformation, 57: 177-189 [DOI: 10.1016/j.jag.2016.12.020] [百度学术]
Yan X, Li Z Q, Luo N N, Shi W Z, Zhao W J, Yang X C, Liang C, Zhang F and Cribb M. 2019. An improved algorithm for retrieving the fine-mode fraction of aerosol optical thickness. Part 2: application and validation in Asia. Remote Sensing of Environment, 222: 90-103 [DOI: 10.1016/j.rse.2018.12.012] [百度学术]
Zhang H S, Li J, Wang T, Lin H, Zheng Z Z, Li Y and Lu Y F. 2018a. A manifold learning approach to urban land cover classification with optical and radar data. Landscape and Urban Planning, 172: 11-24 [DOI: 10.1016/j.landurbplan.2017.12.009] [百度学术]
Zhang H S, Lin H and Wang Y P. 2018c. A new scheme for urban impervious surface classification from SAR images. ISPRS Journal of Photogrammetry and Remote Sensing, 139: 103-118 [DOI: 10.1016/j.isprsjprs.2018.03.007] [百度学术]
Zhang H S, Lin H, Zhang Y Z and Weng Q H. 2015. Remote Sensing of Impervious Surfaces in Tropical and Subtropical Areas. Boca Raton, FL: CRC Press [百度学术]
Zhang H S, Wang T, Liu M F, Jia M M, Lin H, Chu L M and Devlin A T. 2018b. Potential of combining optical and dual polarimetric SAR data for improving mangrove species discrimination using rotation forest. Remote Sensing, 10(3): 467 [DOI: 10.3390/rs10030467] [百度学术]
Zhang H S, Zhang Y Z and Lin H. 2012. A comparison study of impervious surfaces estimation using optical and SAR remote sensing images. International Journal of Applied Earth Observation and Geoinformation, 18: 148-156 [DOI: 10.1016/j.jag.2011.12.015] [百度学术]
Zhang H S, Zhang Y Z and Lin H. 2014a. Seasonal effects of impervious surface estimation in subtropical monsoon regions. International Journal of Digital Earth, 7(9): 746-760 [DOI: 10.1080/17538947.2013.781241] [百度学术]
Zhang Y Z, Zhang H S and Lin H. 2014b. Improving the impervious surface estimation with combined use of optical and SAR remote sensing images. Remote Sensing of Environment, 141: 155-167 [DOI: 10.1016/j.rse.2013.10.028] [百度学术]
Zhong C, Li H, Xiang W, Su A J and Huang X F. 2012. Comprehensive study of landslides through the integration of multi remote sensing techniques: framework and latest advances. Journal of Earth Science, 23(2): 243-252 [DOI: 10.1007/S12583-012-0245-6] [百度学术]